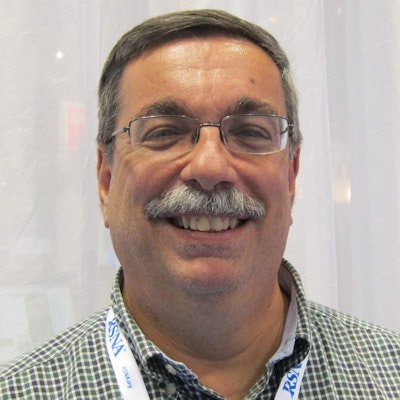
Artificial intelligence (AI) has generated much excitement in radiology, but there are important practical considerations that will affect adoption of the technology. In this new article series, I'll explore several crucial real-world issues that have largely been overlooked amidst all of the hype over radiology AI.
At RSNA 2018, more than 70 vendors displayed a bunch of amazing stuff all dedicated to AI and image interpretation in the Machine Learning Pavilion. Sadly, real-world questions went unanswered, such as how the AI software shown would be integrated with PACS, how it would be paid for by facilities, and whether radiology AI can be used clinically (only seven out of the more than 70 vendors had received U.S. Food and Drug Administration clearance at the time of the meeting). Most questions still remain unanswered.
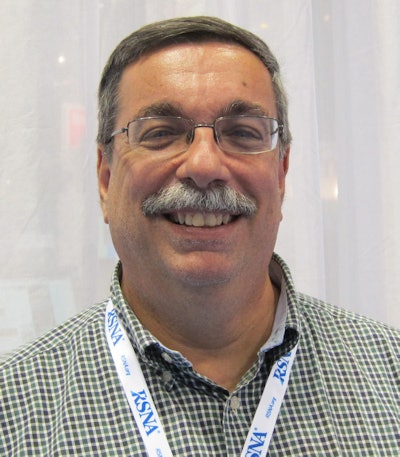
Whether people accept it or not, healthcare in the U.S. is a business. Businesses don't run on altruistic intentions alone. Providing quality patient care is absolutely crucial, but doors don't stay open when there isn't enough money coming in to pay the bills. That's why I'm focusing on reimbursement in the first article of this series.
It's great to hear about how AI can improve outcomes and patient care by shortening length of stay, decreasing readmissions, reducing testing, increasing accuracy, and lowering physician burnout, etc. The reality is, if you can't justify the purchase in ways that those who control the purse strings will accept or get money for it otherwise, the use of AI in an institution just isn't going to fly. Like nearly everything being used in a healthcare setting, AI has to be paid for somehow, either by showing a direct return on investment (ROI) from reimbursement for using the software or indirectly through other savings.
The chief financial officers (CFOs) and others aren't that easy to convince either. That's the real world. Someone also needs to pay for the investments being made in these AI companies -- $5.1 billion in 2018 alone -- so that investors can show an ROI in a reasonable time frame.
A big issue
Reimbursement is a big issue, yet no current procedural terminology (CPT) code currently exists for AI use. Several facilities using breast AI algorithms are filing for reimbursement using CPT codes for computer-aided detection (CAD) for mammography -- codes 77066 and 77067. Others using AI in other applications are filing for reimbursement using CPT codes for 3D processing and advanced visualization -- 76306 and 76307. Is this correct or even legal? I am no reimbursement specialist by any means, but let's just say I wouldn't want to be around when an audit is done on these cases.
Dr. Kurt Schoppe of Radiology Assoc. of North Texas in Fort Worth addressed the question of AI reimbursement in an article published online July 19, 2018, in the Journal of the American College of Radiology. In his article, he shared the hurdles AI software must clear to receive a new CPT code. First, peer-reviewed research in the U.S. would need to demonstrate that the procedure is efficacious and safe. In addition, the procedure would also need to be widely performed by a large number of physicians in the U.S., according to Schoppe.
But even if at least one AI tool could fulfill those requirements, it would then need to be valued by the Relative Value Scale Update Committee (RUC) to get assigned a relative value unit (RVU), he said. These RVUs are recommended based on the amount of physician work and practice expense.
However, "for most AI tools I have seen, there is minimal to no physician work," Schoppe wrote.
Not a direct expense
As for practice expenses, AI software will likely be "bureaucratically mischaracterized" like PACS and will not be considered by Medicare to be a direct expense, according to Schoppe.
"If there is no direct attributable expense on a per-patient basis for the AI software, then it, too, will be an indirect practice expense," he wrote. "That means no direct payments for your accountants to point to for a return on investment."
Ultimately, Schoppe believes that neither government nor private payors will reimburse physicians and hospitals for using AI-based software.
"We will invest in AI software to ensure we are delivering high-quality work, to increase our efficiency, and to simplify clerical type tasks," he wrote. "In this way, paying for AI tools will merely be a cost of doing business like other operational expenses we incur."
In a blog post on April 18, 2018, Dr. Bibb Allen Jr., chief medical officer of the ACR Data Science Institute, added that it's clear there won't be a universal payment scheme for AI in healthcare:
The system is made even more complicated because clearly there will not be a one-size-fits-all payment scheme for reimbursing the use of AI in healthcare. Some algorithms will affect payments to physicians, perhaps making our work either more efficient or more time-consuming as we bring more patient information into our care of complex patients. Some algorithms will improve the overall quality and efficiency of our practices and health systems but cannot be attributed or assigned to a specific service or procedure. While some algorithms may be directly reimbursable by third party payors, many won't be. Finally, all algorithms that are adopted by physicians and our health systems must be proven to provide demonstrable value to patients in a safe and bias-free environment.
He goes on to discuss how AI might fit into the U.S. Centers for Medicare and Medicaid Services (CMS) Quality Payment Program, which includes the Merit-Based Incentive Payment System (MIPS) and Advanced Alternative Payment Models (APMs):
Measures for quality, improvement activities, and advancing care information are reported to CMS by physicians. If certain AI algorithms have documented value and have improved quality to our patients, use of these could potentially at some point be included as MIPS measures. While APMs are much less prevalent in the U.S., algorithms that increase overall efficiency for health systems will be welcome as the medical community strives to do more for our patients at a lower overall cost. When using APMs, assigning and attributing a per-unit cost for an AI algorithm to an individual CPT code will be much less important than ensuring that the algorithm functions in a way that augments the care provided to patients without taking away the common-sense decisions of physicians and patients.
Showing an ROI
Because there isn't a CPT code for reimbursement yet, how can a facility implement AI and show an ROI? By citing indirect savings and hoping that these will show an ROI within a short enough time frame.
One of the most promising AI algorithms in healthcare has been developed by the University of Pittsburgh Medical Center. Its machine-learning algorithm, which is designed to identify patients at the highest risk of rehospitalization within seven and 30 days of discharge, has been able to reduce seven-day readmissions by about 50%, according to an article published online March 4 in Health Data Management.
Developing the software wasn't cheap or quick, requiring a 15-person clinical analytics team and over five years and $100 million to complete, Health Data Management reported. While the investment made by this facility was huge, if the seven-day readmission rate can be reduced by 50%, then that ROI is significant as well.
Even though this was developed by an academic facility, it seems likely that the software will eventually make its way to the commercial marketplace, just as several other products from this institution have. Indeed the amount spent on this single AI product alone is larger than for each of the top 25-funded AI companies. If you eliminate the top six AI firms, the investment made here is more than nearly all the remaining 19 top-funded AI companies combined.
That said, the patient mix and other factors may impact how well the algorithm works in different geographic areas. What works well for one facility might not be the same for others, so how successful the algorithm will be in a variety of settings remains to be proved. It also remains to be seen how many end users will adopt it. Pricing no doubt will be crucial.
AI use cases
Where does the interest in AI lie in radiology? A 2018 report from Reaction Data showed breast imaging, lung imaging, and chest x-ray were the leading use cases for those who planned on employing AI, with 68%, 61%, and 58%, respectively, citing those as key areas. Bone, cardiovascular, and liver imaging constituted the second tier of interest at 38%, 36%, and 34%, respectively, while neural aneurysms (brain) and pulmonary hypertension were planned to be used by 26% and 16% of survey respondents.
Interestingly, breast imaging was far out front among those using AI, leading by a 3-to-1 margin over lung imaging. Meanwhile, AI was utilized in cardiovascular, chest x-ray, and liver imaging applications by 19% of current users, followed by pulmonary hypertension, bone, and others for 13% of users and neural aneurysm by only 6% of users. These numbers are sure to change once AI adoption increases -- especially for lung imaging and chest x-rays. However, the issue of reimbursement becomes even more crucial for chest x-rays due to the low payment for the professional component of the study.
Indeed, paying for AI will make or break the industry. That is why we need to consider paying for AI beyond reimbursement alone. In part 2 of this series, I'll discuss other considerations that may aid in the ROI discussion and share how vendors are charging for AI now and how they may in the future. I'll also offer some guidance on when investors expect to see payback for their investments.
Michael J. Cannavo is known industry-wide as the PACSman. After several decades as an independent PACS consultant, he worked as both a strategic accounts manager and solutions architect with two major PACS vendors. He has now made it back safely from the dark side and is sharing his observations.
His healthcare consulting services for end users include PACS optimization services, system upgrade and proposal reviews, contract reviews, and other areas. The PACSman is also working with imaging and IT vendors developing market-focused messaging as well as sales training programs. He can be reached at [email protected] or by phone at 407-359-0191.
The comments and observations expressed are those of the author and do not necessarily reflect the opinions of AuntMinnie.com.