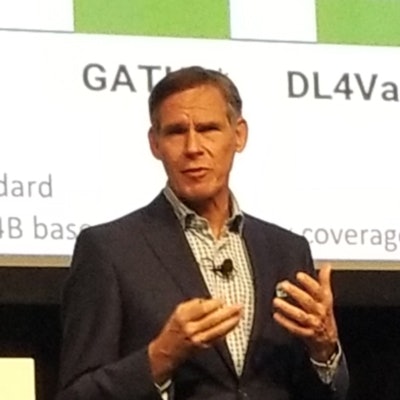
SAN JOSE, CA - The ever-expanding clinical application of artificial intelligence (AI) is changing the face of healthcare -- not only extending the scale of medicine's influence on society but also fostering deeper empathy among clinicians, Dr. Eric Topol said in a March 19 talk at the GPU Technology Conference (GTC).
From the patient to the clinician to healthcare systems, AI and deep learning are affecting all of those involved in medicine, he noted. And physicians must make the most of these tools if they are to be activists who stand up for patients in the years to come. Topol is the founder and director of Scripps Research Translational Institute in La Jolla, CA, and former chair of cardiovascular medicine at Cleveland Clinic.
"As we go forward, human intelligence is probably not going to change very much, but machines are going to get smarter -- very quickly, in fact," he said. "But we need to get smarter; we need to get better. And that's to be more humane. That's what I envision as the output, the end result [of AI in healthcare] ... taking deep phenotyping and using deep learning together to get to deep empathy and concern."
AI personalizes medicine
On the patient level, AI is making it possible for individuals to process their own data and be more proactive about their health, he continued. Patients are empowered by existing AI tools, and many that are in the pipeline, to personalize their nutrition and well-being.
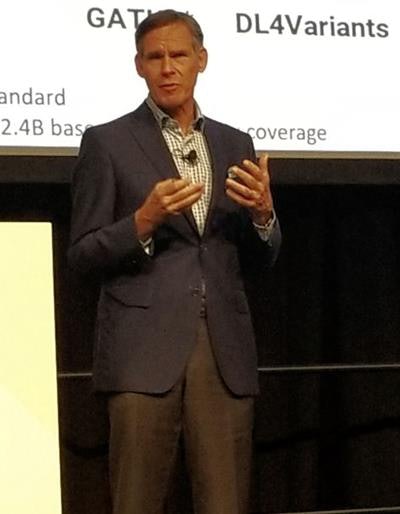
Using himself as a precedent, Topol pointed to an echocardiogram of his heart that he had captured with a portable, smartphone-compatible ultrasound device. He stated that the device offered imaging quality nearly matching that of a $350,000 ultrasound machine, and it allowed him to examine video loops of heart flow in real-time. What's more, the device could potentially enable whole-body visualization in a matter of minutes, relying on little more than a smartphone and an ultrasound probe.
Intrigued by this notion, Topol tried capturing a "medical selfie" of his entire body using the technology. His ultrasound medical selfie revealed a dilated left kidney, which, along with his abdominal pain, suggested to him that he likely had a kidney stone. When he brought this information to an emergency physician, the physician sent Topol to get a CT scan (costing roughly $3,000) that just confirmed his initial supposition.
The point is not that people should start diagnosing themselves but rather that AI is going to allow clinicians to feed all of these data into neural networks, and the resulting, processed information is going to transform the way clinicians assess and share patient health information, Topol explained.
"These AI tools have started to guide the doctor who is uninitiated [using the tools] on how to do the ultrasound and to coach [the doctor] to get a better acquisition," he said. "Soon there will be algorithms to interpret the ultrasound anywhere in the body through a smartphone. ... It's remarkable, and it's going to be the future."
Deep medicine
Numerous studies have shown that deep neural networks can improve the efficiency of clinical tasks, including evaluating cardiac function on cardiograms, classifying skin cancers on photos, and identifying easily missed colonic polyps on colonoscopies, according to Topol. More so than other physician specialties, radiology can benefit from the increase in speed and accuracy that AI lends to image interpretation.
In one example, Topol highlighted the capacity of an AI algorithm to detect clinically relevant findings on x-rays and other medical images approximately 10,000 times faster than a typical reading by a radiologist, at a cost of $1 per scan. Yet he also stressed that such algorithms best serve to augment the performance of radiologists rather than replace their work.
"We're not going to get to the point where all medical diagnoses will not require human backup, ever," he said. "But we will get to a point where some of them -- like a sore throat, ear infection, or skin rash -- can be done algorithmically, both for diagnosing and the recommendation of treatment."
AI appears to be headed in the direction of virtual medical assistance, where clinicians can enter multimodal data into algorithms that can then provide feedback to those who want to have AI guidance for managing diseases, Topol noted. Perhaps the greatest impact in the near term for deep learning is going to be keyboard liberation. Even a single minute away from the keyboard can result in huge savings in time, allowing physicians to spend more time being doctors rather than data clerks.
"When you have patients doing more, getting data, and giving feedback -- and physicians avoiding errors, keyboards, and burnout -- you get this flywheel effect," he said. "You get to this level of deep medicine ... the human bond -- the presence, the trust, and the intimate relationship that used to be there some decades ago."
AI as science
Following his talk, Topol invited Dr. Richard White, chair of radiology at the Ohio State University College of Medicine, to provide insight into the integration of AI in healthcare from the radiologist's perspective.
"I think we're going to see AI as our systems and as a way to help eliminate errors," White said. "I think we'll incorporate data curation into the act of reading so that we're constantly improving models as we go."
What often holds back AI research in healthcare is the limited number of annotated datasets available, he added. Developing effective algorithms and incorporating them into the radiology workflow is a dynamic process that is going to require access to robust data from different sources. If we want to get there faster, we need to invest in building and labeling these datasets.
"We may entertain having boutique datasets to answer specific questions, and that can open up some opportunities for novel data augmentation," White noted. "Ultimately, I think AI is a science and not a product."