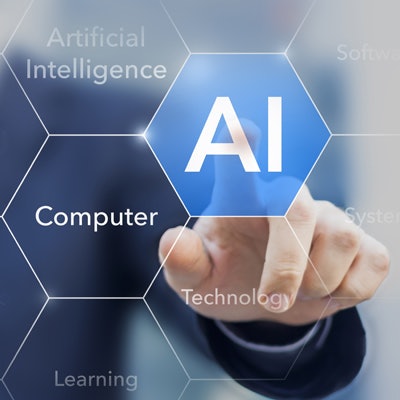
Can an artificial intelligence (AI) algorithm interpret screening mammograms as accurately as expert radiologists can? Yes, but an approach that blends analysis from both AI and radiologists yields the best performance, according to research published March 21 on arXiv.org.
After training a deep-learning algorithm on more than 200,000 screening mammograms, researchers led by first author Nan Wu of New York University (NYU) and senior author Krzysztof Geras, PhD, of NYU Langone Health, found that a hybrid approach including both expert radiologists and a neural network outperformed either method individually.
This suggests that the "use of such a model could improve radiologist sensitivity for breast cancer detection," the authors wrote.
The researchers trained their deep convolutional neural network (CNN) on 229,426 digital screening mammograms from 141,473 patients. Of these studies, 5,832 had at least one biopsy performed within 120 days of the mammogram; 985 had malignant findings, 5,556 had benign results, and 234 had both malignant and benign findings.
Importantly, they trained the algorithm using two different types of image labels. Breast-level labels indicated a benign or malignant finding in each breast, while pixel-level labels then showed the location of the biopsied malignant and benign findings as "heat maps" on the image.
Next, they compared the model's performance with that of human radiologists in a reader study involving 14 readers: a resident, a medical student, and 12 attending radiologists with experience ranging from two to 25 years. Each of the readers read 740 exams from the test set, including 368 exams randomly selected from the biopsied subpopulation and 372 exams randomly selected from those that weren't matched with any biopsy. For each breast, the readers estimated the probability of malignancy on a scale of 0% to 100%.
In addition to comparing the results from the human readers and the deep-learning algorithm, the researchers also evaluated the accuracy of a human-machine hybrid approach -- a linear combination of predictions from the radiologist and the model. Performance was assessed using the receiver operating characteristic (ROC) curve -- a summary of the trade-off between the true-positive rate and false-positive rate -- as well as the precision-recall curve, which summaries the trade-off between the true-positive rate and positive predictive value using different probability thresholds.
Performance in reader study | ||||
Human readers | Deep-learning model | Human-deep-learning hybrid (mean) | ||
Mean | Range | |||
Area under the curve (AUC) | 0.778 | 0.705-0.86 | 0.876 | 0.891 |
Precision-recall AUC (PRAUC) | 0.364 | 0.244-0.453 | 0.318 | 0.431 |
"These results suggest our model can be used as a tool to assist radiologists in reading breast cancer screening exams and that it captured different aspects of the task compared to experienced breast radiologists," the authors wrote.
The researchers would now like to test the utility of their model in real-time reading of screening mammograms. Also, a "clear next step would be predicting the development of breast cancer in the future -- before it is even visible to a trained human eye," the authors wrote.
They have made their model publicly available for other research groups on GitHub.