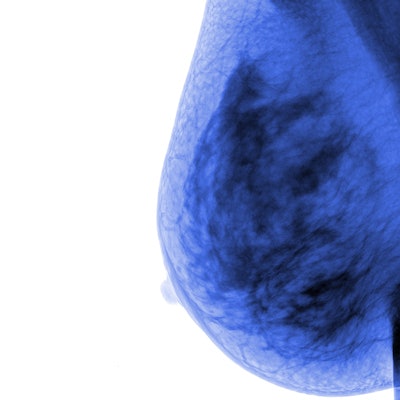
By analyzing subtle imaging patterns on screening mammograms that may portend future cancer, a deep-learning algorithm can beat current breast cancer risk-assessment methods that only assess traditional risk factors such as breast density and family history, according to research published online May 7 in Radiology.
Researchers from the Massachusetts Institute of Technology (MIT) in Cambridge and Harvard Medical School in Boston used nearly 90,000 full-resolution screening mammograms and cancer outcomes data to train a deep-learning algorithm. In testing, their model significantly outperformed a risk-assessment tool commonly used in clinical practice. Importantly, the algorithm was also equally accurate across women of diverse ages, races, and family histories.
"Deep-learning models based on mammography have the potential to replace traditional risk models and support accurate personalized screening and prevention strategies," said lead author Adam Yala, a doctoral candidate at MIT.
Seeking better risk models
Understanding who's at risk of developing breast cancer is a key component of earlier detection and better outcomes. For decades, researchers have sought to develop better breast cancer risk-assessment methods, largely driven by human intuition of risk factors such as age, breast density, family history, and hormonal information, according to Yala.
"Despite these efforts, these models still aren't very accurate at the individual level," he told AuntMinnie.com.
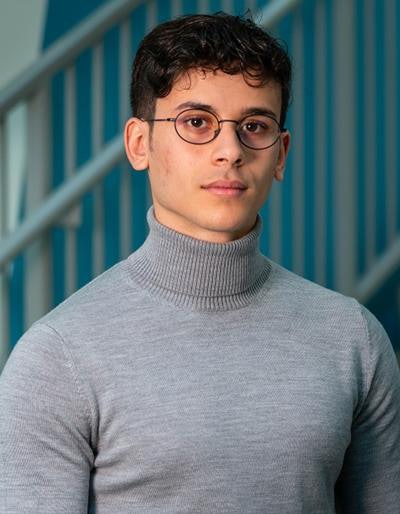
In the hope of achieving better results, Yala; senior author Regina Barzilay, PhD, of MIT; Dr. Constance Lehman, PhD, of Harvard; and colleagues developed a deep-learning algorithm to analyze mammograms to assess breast cancer risk within five years. Rather than relying on manual identification on mammograms of patterns that drive future cancer, however, their model deduced the patterns on its own from a large dataset of mammograms, according to Yala.
The researchers trained and tested a deep convolutional neural network on 88,994 consecutive screening mammograms acquired on mammography systems from Hologic in 39,571 women between January 1, 2009, and December 31, 2012. Of these mammograms, 71,689 were used for training and 8,554 were utilized for validation. The remaining 8,751 were set aside for testing. Cancer outcomes for all patients were gathered from a regional tumor registry.
The team developed and assessed three different risk-assessment methods:
- A logistic regression statistical model based on traditional risk factors
- A deep-learning model based on mammograms alone
- A hybrid deep-learning model that uses both mammograms and traditional risk factors
The researchers then compared the performance of these techniques with version 8 of the Tyrer-Cuzick model, a traditional risk-assessment method that incorporates breast density data.
More accurate, equitable
The researchers found that the deep-learning models were significantly more accurate and equitable than existing approaches for predicting breast cancer risk.
Performance of 4 models for predicting breast cancer risk | ||||
Tyrer-Cuzick model | Logistic regression model based on traditional risk factors | Deep-learning model based only on mammogram | Hybrid deep-learning model based on mammogram and risk factors | |
Area under the curve (AUC) for all women | 0.62 | 0.67 | 0.68 | 0.70 |
AUC for white women | 0.62 | 0.66 | 0.69 | 0.71 |
AUC for African American women | 0.45 | 0.58 | 0.69 | 0.71 |
Portion of breast cancers categorized in highest risk decile | 18% | 31% | 22% | 31% |
The increase in AUC for the hybrid deep-learning model for all women was statistically significant in comparison with the Tyrer-Cuzick model (p < 0.001) and the logistic regression model (p = 0.01). In contrast with the Tyrer-Cuzick model, the deep-learning model was also equally accurate in both white and African American women.
In other key findings, patients with nondense breasts who were classified as high risk by the deep-learning model had a cancer rate 3.9 times higher than women with dense breasts judged to be low risk by the deep-learning model. This shows that there's more information in a mammogram than just the four categories of breast density, according to the researchers.
"The model learned to pick up on subtle patterns in the breast tissue that are precursors to malignancy and is significantly more accurate than existing approaches," Yala said.
Accurate risk assessment
The researchers noted that although the hybrid deep-learning model was the best overall, the deep-learning model based only on mammograms also outperformed the Tyrer-Cuzick method, therefore enabling accurate risk assessment when traditional risk-factor information isn't available.
"This can be especially beneficial to patients who do not know their family history of breast or ovarian cancer," the authors wrote. "In addition, image-only [deep-learning] risk assessment could be rapidly implemented into breast imaging screening programs, with patient risk automatically assessed from the mammogram alone."
The researchers are in the early stages of piloting their deep-learning model clinically and are pursuing collaborations to externally validate their models in diverse patient populations, Yala said. They have made the trained model and code available on their team's website, Learning to Cure.
"Our goal is to make these advancements part of the standard of care and support earlier detection than was previously possible," Yala noted. "To this end, we're excited to further validate our model across diverse populations and to implement it clinically. We are also eager to apply our approach to other diseases where early detection and better risk estimation can play a crucial role, like pancreatic cancer."