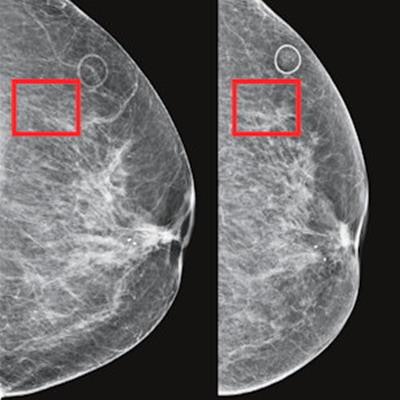
An artificial intelligence (AI) algorithm called Mirai can outperform other risk-assessment models for predicting future risk of breast cancer from a mammogram, potentially enabling earlier identification of high-risk women, according to research published online January 27 in Science Translational Medicine.
A team of researchers led by Adam Yala, a PhD student in MIT's Computer Science and Artificial Intelligence Laboratory (CSAIL), developed Mirai, a deep-learning algorithm that can estimate a woman's risk of breast cancer across multiple future time points based on analysis of their mammogram and clinical risk factors.
In testing on datasets from the U.S., Sweden, and Taiwan, Mirai demonstrated generalizable performance and was significantly more accurate than the commonly used Tyrer-Cuzick breast cancer risk-prediction model as well as two previously developed deep-learning algorithms.
Following up on earlier work by MIT to apply deep learning for breast cancer risk assessment, the MIT team trained Mirai using 210,819 mammograms from 56,786 patients in the Massachusetts General Hospital (MGH) dataset.
In addition to analysis of the mammogram, Mirai considers patient risk factors such as age, family history, and hormonal factors. But if those aren't available when the mammogram is input into the algorithm, Mirai can predict these risk factors directly from the mammogram.
Mirai consists of four modules. After an image encoder receives and processes all standard mammogram views, an image aggregator module then combines the image information across all views to create a representation of the mammogram. If necessary, a risk-factor predictor module then predicts the patient's risk factors from the mammogram. In the last step, an additive-hazard layer predicts a patient's risk each year over the next five years based on analysis of the mammogram and the patient risk factors.
After validation on 25,644 exams from 7,020 patients, the researchers tested Mirai on a hold-out test set of 28,555 exams from 7,005 patients. They also assessed the algorithm's generalizability using datasets from Karolinska University Hospital in Sweden and Chang Gung Memorial Hospital (CGMH) in Taiwan. The Karolinska dataset included 19,328 exams with 1,413 positive cases from 7,353 patients, while the CGMH dataset comprised 13,356 exams with 244 positive cases from 13,356 patients.
They found that the algorithm maintained its performance on the two external datasets without undergoing any additional training, yielding concordance indices of 0.76 for the MGH test set, 0.81 for the Karolinska test set, and 0.79 on the CGMH test set.
What's more, Mirai's five-year risk predictions yielded areas under the curves (AUCs) of 0.76 for the MGH test set, 0.78 for the Karolinska test set, and 0.79 on the CGMH test set. These five-year AUCs were significantly higher (p < 0.001) than the Tyrer-Cuzick model, as well as two deep-learning models previously developed using the same MGH dataset: Hybrid DL -- a deep-learning model that incorporates analysis of mammograms and patient risk factors -- and ImageOnly DL, a deep-learning algorithm based only on mammograms.
In addition, Mirai more accurately identified high-risk patients than other methods across all datasets.
Mirai AI model vs. other breast cancer risk models | ||||
Tyrer-Cuzick model (Version 8) | ImageOnly DL | Hybrid DL | Mirai AI algorithm | |
MGH test set | 22.9% sensitivity, 85.4% specificity | 32.9% sensitivity, 85.9% specificity | 36.1% sensitivity, 86% specificity | 41.5% sensitivity, 85.6% specificity |
Karolinska test set | n/a | 18.9%, 93.1% specificity | n/a | 26% sensitivity, 93.1% specificity |
CGMH test set | n/a | 24.5% sensitivity, 88.5% specificity | n/a | 37.4% sensitivity, 88.5% specificity |
"We also demonstrated how Mirai could be implemented in current clinical pipelines focused on identifying high-risk patients and showed that it improved over existing risk models such as Tyrer-Cuzick lifetime risk and ImageOnly DL," the authors wrote.
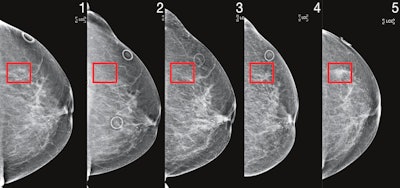
Importantly, Mirai also performed similarly across races, ages, and breast density categories in the MGH test set, as well as across cancer subtypes on the Karolinska dataset.