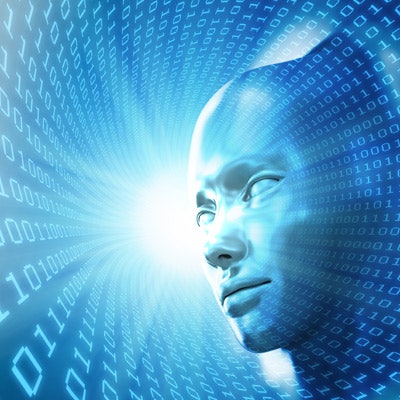
An artificial intelligence (AI) algorithm can improve radiologists' performance for detecting pulmonary nodules on chest CT scans while also speeding up reading times, researchers from China reported in an article published online May 29 in Radiology: Artificial Intelligence.
After training and testing a deep-learning model, a team of researchers from Changzheng Hospital in Shanghai and Infervision Advanced Institute in Beijing found that radiologists detected significantly more lung nodules with the aid of the algorithm than they did during standard interpretations.
"Deep learning shows promise to enhance the identification of pulmonary nodules and benefit nodule management," wrote the authors led by Kai Liu of Changzheng Hospital.
The researchers trained and tested the deep-learning model on 12,754 thin-section chest CT scans that had been retrospectively gathered from multiple hospitals in China, and independently reviewed and annotated by two radiologists who had approximately 10 years of experience in reading chest CT studies. The deep-learning model comprised two convolutional neural networks (CNNs): a DenseNet model extracted image feature maps, while a Faster R-CNN model functioned as the nodule detector. AI software developer Infervision provided hardware and software support for the project.
The deep-learning model had 74% sensitivity when there was one false-positive result per scan. At the cost of specificity, sensitivity improved to 86% when there were eight false positives per exam.
Delving deeper into the data, the researchers found no significant difference in performance for the model related to patient dose, patient age range, or CT scanner manufacturer. The model was, however, more sensitive for solid nodules larger than 6 mm, as well as calcified nodules. It was less sensitive for smaller nodules.
"Such results were consistent with expectations: larger nodules had more abundant features, and the calcified nodules typically had higher signal intensity on CT images," the authors wrote. "Adjusting detection layer resolution of the model may improve the detection of smaller nodules."
To assess the model's ability to enhance manual detection of nodules in clinical situations, the researchers recruited two other radiologists -- each with approximately 10 years of experience -- to read two smaller batches of new cases, initially on their own and then a week later with help from the model. The team assessed performance by calculating area under the curve (AUC).
In the first batch of 123 cases, the deep-learning model led to an increase in sensitivity for both radiologists across all nodule types, according to the researchers. It also enhanced radiologist performance on a per-patient level on the second batch of 148 exams.
Pulmonary nodule detection performance on a per-patient basis | ||
AUC without deep-learning model | AUC with deep-learning model | |
Radiologist 1 | 0.67 | 0.77 |
Radiologist 2 | 0.65 | 0.78 |
What's more, the average reading time per patient dropped for both radiologists, from approximately 15 minutes per patient to approximately five to 10 minutes per patient, according to the researchers.
Because the model's performance didn't depend on multiple external factors, it can be used without restrictions under different imaging conditions, according to the researchers.