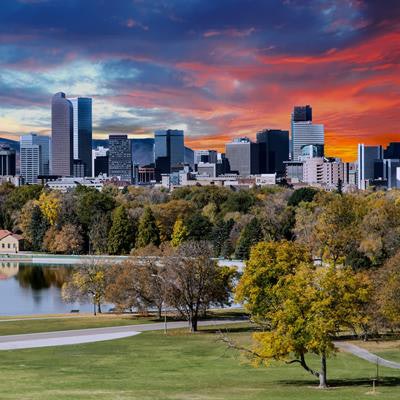
DENVER - An artificial intelligence (AI) algorithm can accurately detect, localize, and classify suspected tuberculosis (TB) on chest x-rays, according to research presented Thursday at the Society for Imaging Informatics in Medicine (SIIM) annual meeting.
A team of researchers led by Jack Luo of McGill University in Montreal trained a deep-learning algorithm that can identify cases of suspicious TB with a high level of classification and localization performance. The researchers believe that their approach would be useful as a triage tool for alerting clinicians and radiologists about TB cases, reducing delays in interpreting these exams.
Although TB is a preventable, detectable, and curable disease, it remains a problem in resource-poor communities in both developing and developed countries. In Canada, the aboriginal communities make up 4% of the population but have 23% of active TB cases, according to Luo.
Timely interpretation of radiographs is hindered by the limited financial infrastructure and human resources available to read those studies, he said.
"The introduction of artificial intelligence into the diagnostic process can reduce the wait for interpretation and improve screening efficiency," Luo added.
Seeking to apply deep learning to triage for suspicious TB exams, the researchers gathered 175 cases of TB-positive frontal chest x-rays and 8,933 negative studies performed at their institution from 2006 to 2017. The positive cases included 156 with infiltrates, 89 with cavitary lesions, and 19 with miliary TB presentations. All positive cases were also manually annotated with bounding boxes for opacity, cavitary, or miliary regions of interest. Of this dataset, 75% of cases were used for training and 25% were set aside for testing.
The researchers then utilized a RetinaNet-based convolutional neural network that was pretrained on the ImageNet database. Additional pretraining was also performed using the RSNA Pneumonia Detection Challenge dataset of 2,500 chest x-rays positive for infiltration.
They then calculated the mean average precision (mAP) to assess the model's localization performance and the area under the curve to evaluate its classification results.
Deep-learning algorithm performance for classifying and localizing TB | |
Deep-learning algorithm | |
Classification of TB presentation | 0.897 |
Overall localization (mAP) | 0.320 |
Luo noted that the team's deep-learning algorithm produced a higher mAP than the winning entry (mAP = 0.255) in the RSNA's recent Pneumonia Detection Challenge.
"[However, it's important to understand] that the results aren't comparable given the differences in the dataset and also the differences in the annotation," he said.
The authors also found that pretraining with the RSNA dataset was beneficial, leading to higher accuracy. In other study results, the algorithm yielded different levels of performance depending on the localization presentation, producing a mAP of 0.384 for infiltrate cases, 0.346 for miliary cases, and 0.253 for cavitary cases.
Luo said the network's performance was limited by the size of minority-class positive cases, especially for cavitary and miliary presentations. The researchers would also like to expand the image dataset by incorporating multicenter databases.
Furthermore, they plan to explore additional alternatives for data augmentation, such as utilizing a generative adversarial network for synthesizing images.