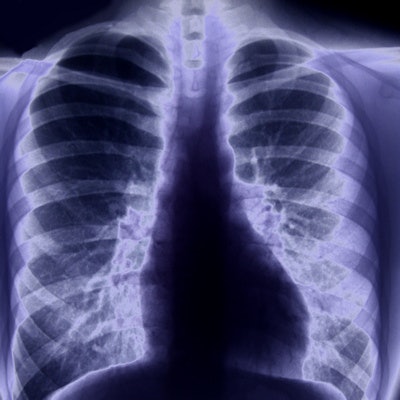
An artificial intelligence (AI) algorithm can accurately identify significant abnormalities on chest x-rays and could improve the sensitivity of radiology residents interpreting these cases in the emergency department (ED), according to research published online October 22 in Radiology.
A team of researchers led by Dr. Eui Jin Hwang of Seoul National University College of Medicine retrospectively analyzed the performance of a commercially available deep-learning algorithm for identifying clinically relevant abnormalities on over 1,000 chest radiographs from their ED. They found that the algorithm yielded an area under the curve of 0.95 and increased the sensitivity of the radiology residents who had initially read the exams.
"The algorithm showed high efficacy in the classification of radiographs with clinically relevant abnormalities from the ED in this ad hoc retrospective review," wrote the authors, who included two employees from AI software developer Lunit. "This suggests that this deep-learning algorithm is ready for further testing in a controlled real-time ED setting."
The researchers used version 4.7.2 of the Lunit Insight for Chest Radiography algorithm to analyze 1,135 consecutive patients who had visited the emergency department between January 1 and March 31, 2017, and had received a chest x-ray. The Lunit algorithm analyzes chest x-rays for the presence of pulmonary malignancy, active pulmonary tuberculosis, pneumonia, and pneumothorax, according to the authors.
They then compared the performance of the algorithm with that of the on-call radiology residents who had actually interpreted the cases in clinical practice. In the event of a discrepant result, the residents then reread the exams with the algorithm's output.
On its own, the algorithm was more sensitive but less specific than the radiology residents. After using the AI software on the discrepant cases, the radiology residents experienced a modest improvement in sensitivity but also a small decrease in specificity.
Performance of AI for abnormalities on chest radiographs | |||
AI algorithm (operating at high sensitivity cutoff) | Radiology residents without AI | Radiology residents with AI | |
Sensitivity | 88.7% | 65.6% | 73.4% |
Specificity | 69.6% | 98.1% | 94.3% |
The improvement in sensitivity (p = 0.003) and decline in specificity (p < 0.001) for the radiology residents from the use of AI were both statistically significant.
Further prospective studies are now necessary to confirm that the use of the algorithm can enhance clinical care and patient outcomes, according to the authors.
In an accompanying editorial, Drs. Felipe Munera and Juan Infante of the University of Miami Miller School of Medicine said they believe that these types of AI tools will improve patient care and benefit radiologists by increasing their efficiency and work satisfaction.
"Furthermore, we believe that such algorithms are likely to improve patient safety if, as the authors suggest, they are employed as triage tools so that cases with a high probability of abnormal findings are prioritized," they wrote. "We look forward to future prospective studies that validate this and other AI algorithms so that they can be confidently introduced into the clinical setting."