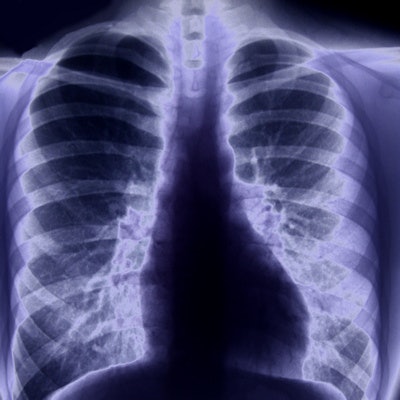
An artificial intelligence (AI) algorithm can identify patients who are at higher risk of long-term mortality by analyzing their chest radiographs, potentially enabling early prevention and lifestyle interventions, according to research published online July 19 in JAMA Network Open.
A team of researchers led by Dr. Michael Lu of Massachusetts General Hospital (MGH) in Boston trained and evaluated a deep-learning algorithm called CXR-risk using data from the Prostate, Lung, Colorectal, and Ovarian Cancer Screening Trial (PLCO) and the National Lung Screening Trial (NLST). The team found that patients identified by CXR-risk as having a very high risk for long-term mortality had a much higher mortality rate than those considered by the model to have low risk.
"This is a new way to extract prognostic information from everyday diagnostic tests," said Lu in a statement from MGH. "It's information that's already there that we're not using, that could improve people's health."
The researchers trained the convolutional neural network to categorize a patient's long-term mortality risk using radiographs and outcomes data from 41,856 patients from the PLCO trial. Next, they tested the algorithm on radiographs from an additional 10,464 patients set aside from the PLCO dataset and from 5,493 smokers included in the screening radiography arm of the NLST. The median patient follow-up was 12.2 years for the PLCO test set and 6.3 years for the NLST test set.
The researchers found that higher CXR-risk scores were associated with higher long-term all-cause mortality rates.
Mortality rates in patients based on CXR-risk scores | |||||
Very low-risk score | Low-risk score | Moderate-risk score | High-risk score | Very high-risk score | |
PLCO test set | 3.8% | 7.8% | 12.7% | 24.9% | 53% |
NLST test set | 2.7% | 3.8% | 6.7% | 9.8% | 33.9% |
The higher mortality in the very high-risk groups compared with the very low-risk groups was statistically significant (p < 0.001).
Furthermore, the association between the CXR-risk score and death remained robust even after the researchers adjusted for the radiologists' diagnostic findings (lung nodules, etc.) and standard risk factors such as patient age, sex, and diabetes status, according to the researchers. Delving further into the data, they found that the algorithm produced similar statistically significant results in both the PLCO and NLST test sets for lung cancer death, noncancer cardiovascular death, and respiratory death.
The study findings suggest that the deep-learning algorithm can stratify a patient's risk of long-term mortality from analysis of their baseline chest radiographs, according to the authors.
"Individuals at high risk may benefit from prevention, screening, and lifestyle interventions," they wrote. "Further research is necessary to determine how this can improve individual and population health."
The researchers also believe that CXR-risk will become even more accurate when combined with other risk factors such as genetics and smoking status.
In an accompanying editorial in JAMA Network Open, Dr. Surafel Tsega of the Icahn School of Medicine at Mount Sinai in New York City and Dr. Hyung Cho of New York City Health and Hospitals noted that deep learning has the potential to augment the judgment of clinicians, potentially leading to improved delivery of care. However, they cautioned that the technology isn't quite there yet.
"The evidence is lurching toward the ability to better predict outcomes, but even the most optimistic artificial intelligence supporters must acknowledge that successful prevention of clinically significant adverse outcomes remains an even more distant possibility," they wrote. "We should not simply be intoxicated by the idea of what we can do but must be clear-headed about what is worth knowing and is worth doing."