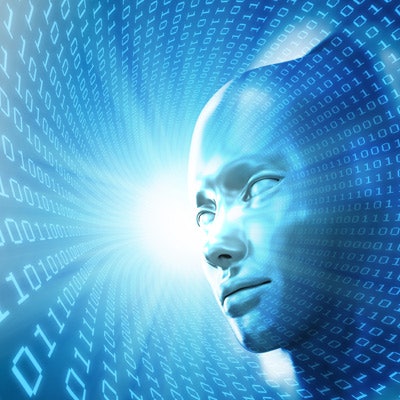
It's not easy to create datasets needed to train generalizable artificial intelligence (AI) algorithms. For the 2019 RSNA Artificial Intelligence Challenge, it took the teamwork of two societies and 60 volunteer neuroradiologists, according to an article published online April 29 in Radiology: Artificial Intelligence.
Although public datasets had been used for the two previous challenges, the RSNA wanted to build its own dataset for the 2019 challenge, which focused on facilitating machine-learning algorithms to assist in the detection and characterization of intracranial hemorrhage on brain CT. To accomplish this goal, the RSNA collaborated with the American Society of Neuroradiology (ASNR) to assemble a multinational and multi-institutional brain hemorrhage CT dataset containing over 874,000 images.
The images were provided by Stanford University in Stanford, CA; Universidade Federal de São Paulo in São Paulo, Brazil; and Thomas Jefferson University Hospital in Philadelphia. All of the images were annotated for each of the five hemorrhage subtypes by a volunteer cadre of 60 members of the ASNR. The volunteers marked each exam as normal or abnormal; abnormal cases were further categorized by hemorrhage subtype.
The resulting RSNA Brain Hemorrhage CT Dataset is the largest public dataset of its kind and contains a large and heterogeneous collection of brain CT studies from multiple manufacturers, institutions, and countries, according to the researchers led by lead author Dr. Adam Flanders of Thomas Jefferson University.
"The dataset is unique, not only in terms of the volume of abnormal images but also the heterogeneity of where they all came from," Flanders said in a statement from the RSNA.
Released under a noncommercial license, the dataset is available for free to the AI research community for noncommercial use and further enhancement, according to the RSNA.
"I was really impressed by the huge volunteer effort and the tremendous worldwide interest in this project," Flanders said. "The dataset we created for this challenge will endure as a valuable [machine-learning] research resource for years to come."
Following the success of the collaboration with a subspecialty society, the RSNA said it plans to use a similar approach for the 2020 challenge. Organizers will team up with the Society of Thoracic Radiology for the competition, which will target improved detection and characterization of pulmonary embolism on chest CT.