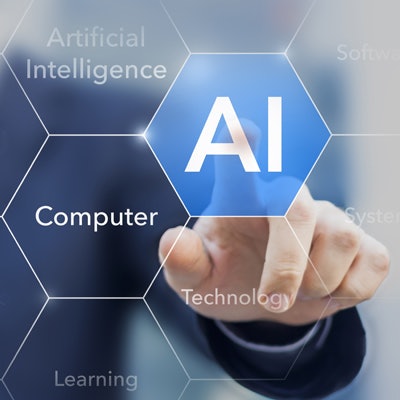
Clinical artificial intelligence (AI) algorithms across multiple disciplines -- including radiology -- are disproportionately trained on patient cohorts from three states: California, Massachusetts, and New York, according to a research letter published September 22 in the Journal of the American Medical Association.
After analyzing the literature for articles published on deep-learning algorithms that perform image-based tasks, researchers from Stanford University found that very few of the studies that included geographically identifiable cohorts utilized training sets from the other 47 states. And 34 states weren't included at all.
"Both for technical performance and for fundamental reasons of equity and justice, the biomedical research community -- academia, industry, and regulatory bodies -- should take steps to ensure that machine-learning training data mirror the populations for which algorithms ultimately will be used," wrote Dr. Amit Kaushal, PhD; Dr. Russ Altman, PhD; and Dr. Curt Langlotz, PhD.
Although AI algorithms have been shown to achieve high accuracy for a variety of image-based diagnostic tasks, these models are also vulnerable to bias, such as when an insufficient amount or diversity of data is used for training, according to the researchers.
They investigated the geographic distribution of training datasets by searching PubMed for peer-reviewed articles published between January 1, 2015, and December 31, 2019. For inclusion in the Stanford analysis, the studies had to utilize at least one U.S. cohort for training, as well as benchmark performance against, or in tandem with, physicians in six clinical specialties: radiology, ophthalmology, dermatology, pathology, gastroenterology, and cardiology.
Unless an alternate method for assembling the training dataset was described, all patient cohorts provided by a hospital or health system were attributed to the home state of the institution, according to the researchers. They also communicated with the corresponding authors of the studies if the cohorts were ambiguous.
They found 74 studies that met the inclusion criteria, including 35 for radiology, 16 for ophthalmology, 11 for dermatology, 8 for pathology, two for gastroenterology, and two for cardiology. Fifty-six (76%) used at least one geographically identifiable cohort, of which 40 (71%) were from either California, Massachusetts, or New York.
Patient cohorts by U.S. state used for training clinical AI algorithms | |
State | Number of studies |
California | 22 |
Massachusetts | 15 |
New York | 14 |
Pennsylvania | 5 |
Maryland | 4 |
Colorado, Connecticut, New Hampshire, and North Carolina | 2 each |
Indiana, Michigan, Minnesota, Ohio, Texas, Vermont, and Wisconsin | 1 each |
The remaining 18 (24%) studies used patient cohorts that were intrinsically geographically heterogeneous or ambiguous, such as those from large studies from the U.S. National Institutes of Health (NIH) or clinical trials that span five or more states, according to the researchers. Overall, 23 multisite cohorts were found, including 13 (57%) from existing NIH studies or consortia, seven (30%) from industry trials or databases, two (9%) from online image atlases, and one (4%) from an academic second-opinion service.
"California, Massachusetts, and New York may have economic, educational, social, behavioral, ethnic, and cultural features that are not representative of the entire nation; algorithms trained primarily on patient data from these states may generalize poorly, which is an established risk when implementing diagnostic algorithms in new geographies," the authors wrote.