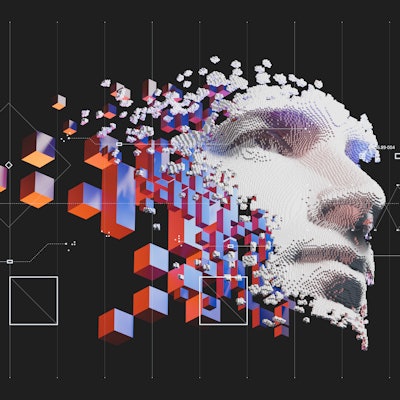
Sure, artificial intelligence (AI) in radiology is cool. But it's not enough to show results in a lab; the technology's real-world impact on efficacy and efficiency also needs to be evaluated, according to a June 25 talk at the virtual annual meeting of the Society for Imaging Informatics in Medicine (SIIM).
It's also crucial to ascertain how radiology AI affects radiologists' perception, cognition, human factors, and workflow, according to Elizabeth Krupinski, PhD, of Emory University.
"We really need to understand and appreciate how technology, no matter how cool it is to develop and implement ... impacts the human user at the end, and after that, how it impacts patient care," said Krupinski during the 2020 Dwyer Lecture at SIIM 2020.
Radiologist burnout is real
Radiology is getting more complex, and fatigue and burnout are real issues among radiologists. AI is being proposed as a way to deal with some of these issues and have radiologists feel like they're on less of a production line, Krupinski said.
Many AI research papers, however, don't include discussion of the relevance or clinical significance of the work, she said.
"I read a lot of studies where they identify a type of image and a type of finding and then slap an AI scheme on it and say, 'Look, we've been able to classify 0.94 under the ROC curve and think this is great,' " she said. "But then when you read it, there's nothing in the significance or the background section that says why they're doing this. Is it a problem right now? Do radiologists have a problem with that particular type of diagnosis or type of image?"
Impact on radiologists
There's also very little serious consideration or study on how AI results are presented during clinical use and how they impact radiologists cognitively, perceptually, and even ergonomically, she said.
"How is it changing what they do and how they do it?" she said.
There also aren't enough studies on the impact of AI on short- and long-term decision making, patient outcomes, and training of radiologists, according to Krupinski said.
"Basically, what's missing in a lot of the AI, deep learning, and some of the technology development -- even hardware -- is a consideration of the users," she said. "And I think that ends up being critically important."
Increased cognitive load?
As an example, many AI schemes are being developed to triage and exclude cases that are definitely normal. This would fundamentally alter the task of the radiologist, as well as change their cognitive and, probably, their perceptual expectations, according to Krupinski.
"The remaining images after the triaging is done are likely going to be harder, they're going to be more complex, and you're going to have increased cognitive load and, potentially, increased stress on the radiologist, the pathologist, or whoever the clinicians are that you're developing these schemes for," she said.
Yes, radiologists will have more time to devote to these exams, but it has yet to be determined if that change will increase radiologist fatigue and if it somehow will fundamentally change the accuracy of their decisions and recommendations, according to Krupinski.
"I think we're really going to have to go through and consider how the tools that we're developing -- all of these cool technologies -- are going to impact that final user and what those implications are then going to be on the information that we provide to other providers, the referring providers, and what we talk about with the patients and so on," she said. "Because I think it's going to fundamentally change things."
A lot of biases, such as anchoring bias and confirmation bias, will also be changed as a result of introducing these types of tools and technologies into the workflow, which could have a significant impact on how radiologists use these tools and their decision-making, she said.
Measuring clinical impact
It's difficult, however, to assess innovations in real-life clinical settings using rigorous methods, she said. Classic studies can be costly and require effort.
But there are options. Some quasi-experimental designs can work, including a variety of non- or partially-randomized designs that blend aspects of effectiveness randomized controlled trials and implementation research, she said.
"The goals of these quasi-experimental designs are to balance the internal and external validities of the studies," she said.
The advantages of these approaches include faster intervention uptake, enhanced acceptability, and a lower cost. They can have biases and errors, but so can practically any type of study design, Krupinski said.
She suggested three types of quasi-experimental designs:
- Prepost with nonequivalent control: In this type of a study, a group with the intervention -- AI -- is compared before and after adoption with a nonrandomized but similar control group that didn't receive AI.
- Interrupted time series: Outcome is measured at consecutive (3-8) time points before and after intervention, all within the same group, which acts as its own control.
- Stepped-wedge: Intervention is rolled out over time at multiple sites. The control groups eventually receive the intervention, acting as their own controls. This enables comparison of the intervention at different institutions or by different groups, facilitating assessment across different practices, institutions, and patient types.
Time-motion studies
Time-motion studies are also important for assessing the potential for time savings from AI, Krupinski said. These can be performed using an external observer, self-reporting by the subject, or with automatic time stamps.
"Usually it's some kind of combination of these three that could be useful for a time-motion study," she said.
Krupinski also noted, however, the potential for these studies to be affected by the Hawthorne Effect -- the alteration of one's behavior due to their awareness of being studied.
"When people are being studied, they know they are being studied and so they rise to the occasion; this is also called the Halo effect," she said. "When you are concerned and you know your people are concerned about whether your productivity is going to increase, you're going to -- consciously or unconsciously -- ramp up your speed or you're going to do what is expected of you. You may change some of your decisions because of the tool."