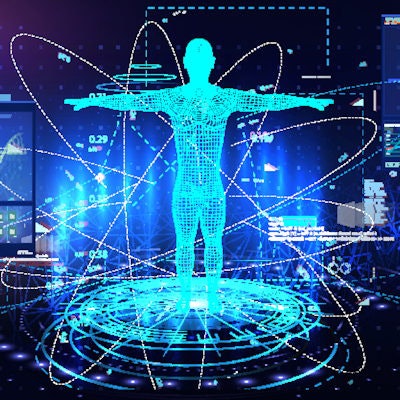
Radiology reports created with help from artificial intelligence (AI) are more accurate and faster to produce than current reporting methods in evaluating the response of advanced cancer to treatment over time, according to research presented at the recent Conference on Machine Intelligence in Medical Imaging (C-MIMI).
An AI-assisted method called AI Mass that integrated graphs, tables, and key images yielded quicker reports with much fewer major errors, according to a research team led by Dr. Brian Allen of Duke University Medical Center. Researchers found that the AI method was also better received by both radiologists and oncologic providers in the study.
"AI-assisted imaging evaluation and reporting of advanced cancer longitudinal response is overwhelmingly more effective than the current practice with manual measurements and text-based reports," Allen said.
He shared the research findings during a scientific session at C-MIMI 2020, which was held by the Society for Imaging Informatics in Medicine (SIIM).
Assessing treatment response
Patients with advanced cancer often undergo repeat imaging of the chest, abdomen, and pelvis to evaluate treatment response. In clinical practice, radiologists evaluate these images and dictate multiple text-based reports. This approach is problematic, however, according to Allen.
"There's inconsistency between radiologists [and] there are multiple manual steps that are prone to errors and are inefficient," he said. "And these text-based reports lack longitudinal data and percent changes in tumor size. Our thought was that an artificial intelligence-guided workflow would overcome these deficiencies and lead to a single patient-level report with a graph, table, and key images."
The researchers sought to perform a multi-institutional, retrospective study to compare the effectiveness of current radiology reporting practice versus AI Mass, an AI-assisted method in advanced cancer longitudinal response evaluation. The AI software used in the study was developed by co-author Dr. Andrew Smith, PhD, of the University of Alabama at Birmingham Medical Center, who is also CEO and owner of AI technology developer AI Metrics.
The study cohort consisted of 120 consecutive adult patients with measurable advanced cancer of any type treated with systemic therapy. All patients had a baseline exam and up to two follow-up exams. The researchers recruited 24 radiologists from 21 institutions, and each read 20 exams per time point with each reporting method. All exams were read in triplicate. Twenty oncologic providers from seven institutions also participated in the study.
In their current practice, radiologists evaluate images using a U.S. Food and Drug Administration (FDA)-cleared web viewer and dictated reports using their normal speech recognition software and reporting style, Allen said. Readers were asked in the study to categorize the intent of their conclusion -- separately from their impression -- as either complete response, partial response, stable disease, or progressive disease.
AI-assisted reports
With the AI-assisted method, radiologists evaluated the images using version 0.5 of AI Mass, a web-based AI-assisted viewer that provides guided workflows and automated calculation of tumor burden and response categorization, according to Allen. Three AI algorithms are utilized to assist with tumor measurement, labeling, and tracking over time.
"The AI works anywhere in the body, and the measurements and labeling are easy to edit," Allen said. "The radiologist never has to take his or her eyes off the images to check for any dictation errors. All measurements and annotations are automatically stored."
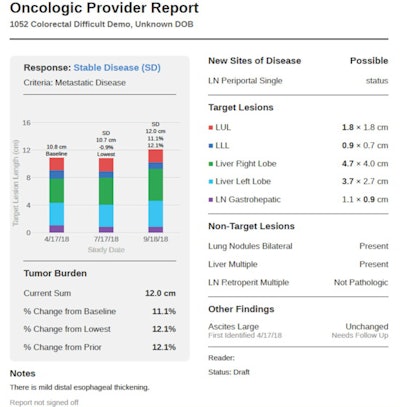
The software's "AutoPilot" feature uses embedded AI to rapidly locate all previously measured tumors to aid in assessing treatment response, he said. At the conclusion of the interpretation, the AI-assisted reports are immediately available, and they include a longitudinal graphical report, a table, and key images. Percent changes in tumor burden are included.
More accurate results
The AI Mass-assisted method performed significantly better for the study's primary measure of accuracy, which was defined as percent agreement between the dictating radiologist and the oncologic provider for the patient's final treatment response category, according to Allen. The researchers also found that the AI-assisted reports had far fewer major errors, which were defined as measurement data transfer errors of ≥ 5 mm, a major discrepancy between the findings and conclusion, laterality errors, or unclear language that markedly limits report interpretation.
Performance of AI Mass reporting vs. current reporting practice | |||
Current reporting practice | AI Mass-assisted reports | Improvement | |
Accuracy of full reports | 73% | 91% | 25% |
Major errors /patient | 27.5% | 0.3% | 99% |
Radiology reading time | 18.7 minutes | 9.8 minutes | 48% |
Radiology interobserver agreement on final report | 62 of 120 (52%) | 90 of 120 (75%) | 45% |
In a survey of the 24 radiologists performed after the study, 88% judged the AI method to be significantly better. In addition, 96% preferred the AI method and 92% believed that patients will prefer AI-assisted reports, according to Allen.
Oncologic providers also approved of the AI-assisted reports. All 20 indicated that the AI reports are significantly better and that they preferred the AI reports. In addition, 90% believed that patients would prefer the AI reports, Allen said.