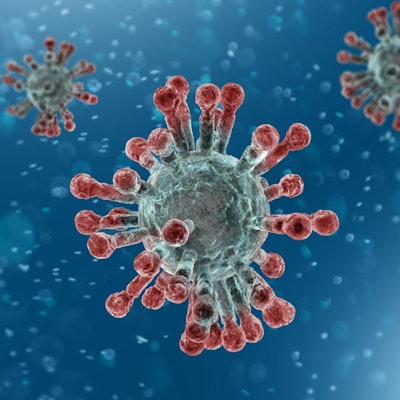
An artificial intelligence (AI) algorithm can predict how COVID-19 cases will progress based on analysis of baseline chest x-rays, offering potential to help in deciding how to triage these patients, according to a study posted online May 5 as a preprint on medRxiv.org.
After learning to detect changes in images on chest radiographs associated with a worsening or improving patient outcome, a deep-learning algorithm trained by a team of researchers in Canada was able to accurately predict outcomes based on an initial chest x-ray of a patient with COVID-19 in over 80% of test cases.
"[Chest x-ray] deep-learning features show promise for classifying the disease trajectory," wrote co-first authors Simon Duschesne, PhD, and Daniel Gourdeau, senior author Dr. Nathalie Duschesne of the Université Laval in Quebec, and colleagues. "Once validated in studies incorporating clinical data and with larger sample sizes, this information may be considered to inform triage decisions."
Setting out to develop a deep-learning algorithm to extract features from chest x-rays to track and predict the radiological evolution of the disease, the researchers first used the CheXnet open chest x-ray dataset to train and validate a repurposed deep-learning algorithm to extract features that were mapped to radiological labels. When tested on CheXnet cases, the algorithm yielded areas under the curve that varied from 0.71 to 0.93 for identifying seven imaging features (enlarged cardiomediastinum, cardiomegaly, lung opacity, edema, consolidation, pleural effusion, and atelectasis), as well as pneumonia.
Next, they tested the algorithm's performance on 60 pairs of sequential chest x-rays from 40 COVID-19-positive patients collected from two open-source datasets from the Italian Society for Medical and Interventional Radiology and Mila. Based on the evaluation of radiological evolution ascertained from images and reports, these pairs of anteroposterior x-rays were first classified by the researchers into one of three categories: worse, stable, or improved.
After computing the differences in the deep learning-calculated probabilities of findings between the initial and the second study, the researchers found that three imaging features -- consolidation, lung lesion, and pleural effusion -- and pneumonia all had significantly different features between the "worse" and "improved" categories (p < 0.05). Next, they created a logistic regression model to determine if these deep-learning features could be used to predict future outcomes based on the patient's initial x-ray.
The algorithm yielded 82.7% accuracy for predicting if a patient's outcome would be "worsening" or "improved."
The researchers concluded that their results were sufficiently convincing to warrant further consideration of incorporating deep-learning features into a clinical prediction rule for supporting triage decision-making by clinicians. However, decisions to institute mechanical ventilation shouldn't rely only on prognostic decision rules, they said.
"Shared decision-making integrating the best available prognostic models, clinician experience, and patient values and preferences about life-sustaining therapies will also be paramount in making these very difficult decisions," the authors wrote. "Depending on the phase of the COVID-19 viral pandemic, decisions may unfortunately only be based on prognosis, the ethical principle of social justice, and availability of mechanical ventilation."