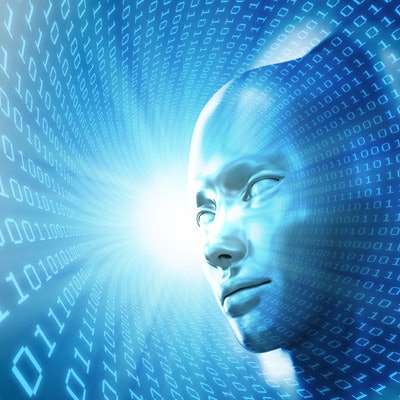
Artificial intelligence (AI) algorithms trained with more cases from one gender can yield biased computer-aided diagnosis applications that perform worse on imaging exams involving the other gender, according to research published online May 26 in the Proceedings of the National Academy of Sciences.
A team of researchers led by Agostina Larrazabal and Nicolás Nieto of National University of Litoral and the National Research Council of Argentina (CONICET) in Santa Fe, Argentina, trained three different neural network architectures to detect 14 thoracic diseases on chest x-rays using various ratios of male and female cases from two popular public image datasets.
They found that the AI algorithms trained with more cases of one gender consistently underperformed when tested on datasets consisting of more studies from the opposite gender.
"This raises the alarm for national agencies in charge of regulating and approving computer-assisted diagnosis systems, which should include explicit gender balance and diversity recommendations," the authors wrote. "We also establish an open problem for the academic medical image computing community which needs to be addressed by novel algorithms endowed with robustness to gender imbalance."
To assess how gender imbalance in medical imaging datasets would impact algorithm performance, the researchers trained three different types of convolutional neural networks (CNNs) -- a Keras implementation of the DenseNet-121; ResNet, and Inception-v3 -- using various male/female gender (0%/100%, 25%/75%, and 50%/50%) ratios in training sets derived from the U.S. National Institutes of Health's Chest-Xray14 and Stanford University's CheXpert databases.
They found a consistent and statistically significant decrease in performance when using male patients for training and female patients for testing, and vice versa. Even with a 25% to 75% gender ratio, all three models produced a significantly lower average performance across all diseases for the minority gender class in comparison with algorithms trained with a gender-balanced dataset, according to the researchers.
"Our results show that using gender-imbalanced datasets to train deep learning-based CAD systems may affect the performance in pathology classification for minority groups," the authors wrote.
The researchers also found that using a gender-balanced dataset yielded comparable statistical performance for each gender compared with algorithms that had an extremely unbalanced dataset for the same gender, according to the researchers.
"Altogether, our results indicate that diversity provides additional information and increases the generalization capability of AI systems," they wrote. "Thereafter, it also suggests that diversity should be prioritized when designing databases used to train machine learning-based CAD systems."
The researchers noted that it's well known that CNNs tend to learn representations that are useful to solve the task that they are being trained for.
"When we go from male to female images (or vice versa), structural changes in the images appear, leading to a change in data distribution which explains the decrease in performance," they wrote. "Algorithmic solutions to such 'domain adaptation' problems should be engineered, especially in cases when it is difficult to obtain gender-balanced datasets [e.g., Autism Brain Imaging Data Exchange (ABIDE)]."
Further results can be found on the researchers' GitHub page.