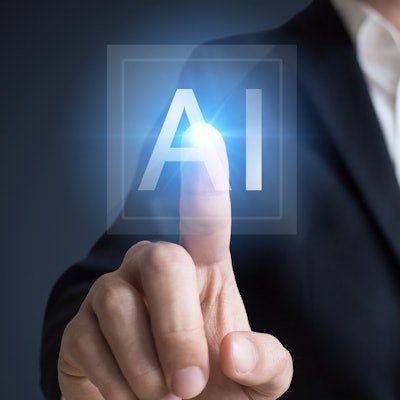
Greater involvement by radiologists and more help from data scientists are needed to improve the performance of artificial intelligence (AI) algorithms and expand their use in clinical practice, a leading Spanish expert told ECR Online registrants on Thursday.
Interactive, continuously learning models can help facilitate radiologist involvement, according to Dr. Luis Martí-Bonmatí, PhD, chairman of the radiology department and director of medical imaging at La Fe University and Polytechnic Hospital in Valencia.
"To improve the prediction accuracy of the machine-learning or neural network solution we have to involve radiologist-humans providing, through trial and error, continuous feedback to the machine-learning model," he said. "So at the end, the predictions will be much better."
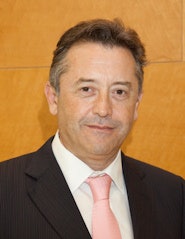
This approach will essentially result in a personalized computer-aided tool in which machines and humans work together to develop lifelong, continuously adaptive systems, said Martí-Bonmatí, who is also the co-founder of AI software developer Quibim and editor in chief of Insights into Imaging.
"Because at the end, the real world is much larger than what we can have from the training and testing models," he said. "And that's why we need this continuous learning to have a much better [result]."
Another challenge to clinical adoption of AI is the predominance of "weak" AI, or algorithms that focus only on one narrow task, such as prostate segmentation, Martí-Bonmatí said.
"We need to have a solution that will ensemble all of those AI partial solutions and really give radiologists a final end-to-end aspect of what we are looking for," he said. "As an example, we want to link the different AIs for organ segmentation, tumor extraction and classification, aggressiveness definition, phenotyping, and treatment allocation."
Creating ensembles of neural networks can help radiologists in the real-world environment by enabling discoveries of unexpected outcomes, as well as yielding opportunistic screening, phenotyping, prediction, and prognosis assessment, according to Martí-Bonmatí.
However, building those types of models will require data scientists working in radiology departments, he said.
"These professionals work on the feature extraction, data integration, data curation, and data mining," he said. "These [areas] are where radiologists need the help of data scientists."
Data scientists can help in constructing training and validation datasets for algorithms, as well as in calculating performance metrics and providing external validation on data from different centers and scanners, according to Martí-Bonmatí.
"So data scientists are helping radiologists in these many aspects of the methodology that we need to implement artificial intelligence in clinical practice," he said.
Data scientists can help radiologists with tasks such as defining variables in databases and employing common data elements, as well as delineating the technology and methodology to be used throughout the AI project. They can also assist in data acquisition, curation, and validation, including continuously reviewing the data, he said. What's more, data scientists can be responsible for storing data in a safe and structured manner, as well as data and modeling and mining, he said.
"We do need to have AI implemented into clinical practice," he said. "We already have so many different solutions. They will be much better tomorrow, and to do that we need not only data scientists working in the radiology departments but also scientists and researchers, like my team."