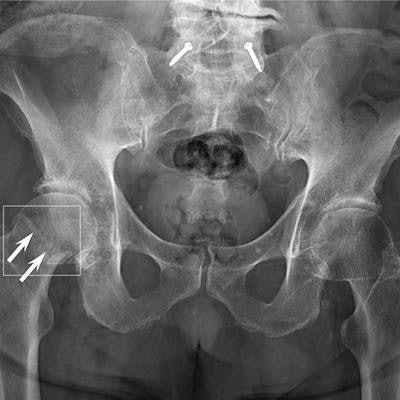
An artificial intelligence (AI) algorithm can improve the detection of trauma fractures on x-ray by radiologists and nonradiologists without lengthening reading time, according to a study in the March issue of Radiology.
Researchers from Boston University (BU) and colleagues at AI software developer Gleamer.ai evaluated a model developed by the vendor based on a dataset of 60,170 radiographs of patients with trauma from 22 institutions. In a test of the model in a dataset representing 480 patients, the model improved fracture detection by radiologists and six other types of readers by 10.4% and cut reading time by 6.3 seconds per patient.
"Radiographic artificial intelligence assistance improves the sensitivity, and may even improve the specificity, of fracture detection by radiologists and nonradiologists involving various anatomic locations," wrote Dr. Ali Guermazi, PhD, professor of radiology and medicine and director of the BU's Quantitative Imaging Center.
Missed fractures on radiographic images are not an uncommon problem in the setting of acute trauma, according to the authors. Moreover, missed fractures are one of the most common causes of diagnostic discrepancies between initial interpretations by nonradiologists or radiology residents and the final read by board-certified radiologists, the authors wrote.
In this study, Guerzani and colleagues assessed the impact of AI assistance on the diagnostic performances of 24 physicians in six subgroups: radiologists, orthopedists, emergency physicians, emergency physician assistants, rheumatologists, and family physicians (four of each).
The dataset included at least 60 examinations for each of the following anatomic regions: foot and ankle, knee and leg, hip and pelvis, hand and wrist, elbow and arm, shoulder and clavicle, rib cage, and thoracolumbar spine. When the AI model's "confidence level" for identifying fractures was surpassed, it highlighted regions of interest with a white square box on the radiograph.
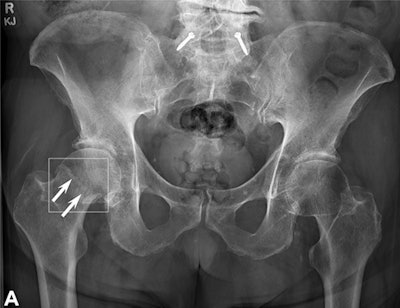
Two experienced musculoskeletal radiologists with 12 and eight years of experience respectively established ground truth by independently interpreting all examinations without clinical information. Only acute fractures were considered to be a positive finding in the study.
All x-rays were independently interpreted by 24 clinicians from multiple institutions in the U.S., including both in-training and board-certified physicians with variable years of experience (2 to 18 years) in radiographic interpretation for fracture detection. All readers were presented, in random order, the 480 radiographic examinations of the validation dataset twice -- once with the assistance of AI software and once without the assistance, with a minimum washout period of one month.
AI-assisted radiographic reading by the six types of readers overall showed a 10.4% improvement of fracture detection sensitivity (75.2% vs. 64.8%; p = 0.001 for superiority) without specificity reduction, the authors found.
In addition, AI assistance shortened the radiograph reading time by 6.3 seconds per patient (p = 0.046). The improvement in sensitivity was significant in all locations (delta mean, 8.0%-16.2%; p = 0.05) but shoulder and clavicle and thoracolumbar spine (delta mean: 4.2% and 2.6%; p = 0.12 and 0.52).
"Artificial intelligence assistance for searching skeletal fractures on radiographs improved the sensitivity and specificity of readers and shortened their reading time," the authors wrote.
The researchers noted that they used an external multicenter dataset from the U.S., including multivendor x-ray systems not related to the development set, which came from Europe. This ensures that the model's performance can be more easily generalized to other clinical settings.
"Our AI system can interpret full-size high-spatial-resolution images, including multiple radiographic views in a patient, and can be integrated into picture archiving and communication systems used in daily clinical practice," the authors wrote.
In an accompanying editorial, Dr. Thomas Link, PhD, and Valentina Pedoia, PhD, musculoskeletal radiologists at the University of California, San Francisco, highlighted several strengths of the study but suggested that at this stage, it is still premature to use AI as a standalone interpretation tool without radiologist readings as well.
The study stands out in terms of complexity and number of physician readers, Link and Pedoia wrote, yet the results highlight a recurrent problem of AI studies -- namely, the ground truth.
"In many studies, the ground truth is based on readings by 'expert' radiologists. But this is not a ground truth, per se. Instead, it is a hypothetical concept used for convenience," they stated.
Critical review and better study designs are required to develop optimized and scientifically grounded AI algorithms, Link and Pedoia concluded.