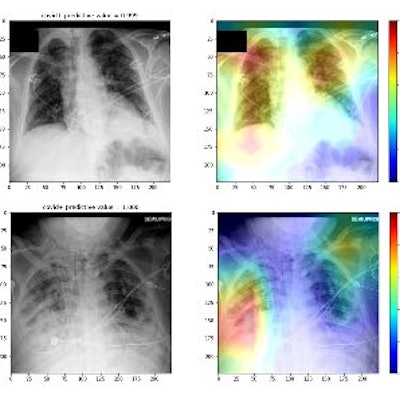
Artificial intelligence (AI)-based tools have not yet reached full diagnostic potential in COVID-19, and underperform compared to radiologist predictions, according to a study published June 1 in Radiology: Artificial Intelligence.
A U.S. group conducted a prospective observational study across 12 hospitals to evaluate the real-time performance of an AI model to detect COVID-19 on patient chest x-rays. While the model appeared promising when tested on a publicly available dataset, it was inadequate for diagnosing patients who presented with COVID-19 symptoms, the researchers found.
"It may be impossible to develop a model solely based on [chest x-ray] findings alone to differentiate between participants with COVID-19 and non-COVID-19 diagnoses," wrote senior co-authors Erich Kummerfeld, PhD, and Dr. Christopher Tignanelli of the University of Minnesota's Institute for Health Informatics in Minneapolis.
Reverse transcription polymerase chain reaction (RT-PCR) tests are the mainstay of COVID-19 diagnosis. Yet early in the pandemic, access to RT-PCR tests was a bottleneck in testing, and this spurred efforts to develop AI-based models for analyzing medical images.
One recent literature review identified 62 AI diagnostic and prognostic imaging models for COVID-19 and noted that none of the 45 diagnostic models published included a prospective real-time validation of model performance, according to the authors.
"To our knowledge, other prospective observational studies to investigate the real-world performance of an AI model for COVID-19 diagnosis based on [chest x-ray] findings alone have not yet been performed," they wrote.
In this study, the group, which included investigators at Emory University in Atlanta and Indiana University in Indianapolis, used 91,020 chest x-rays in all for model training and external and real-time validation.
First, the researchers tested the model using a publicly available COVID-19 dataset from the University of Minnesota of 1,777 patients with RT-PCR-confirmed moderate or severe disease and 5,228 participants who tested negative. On this dataset, the AI model detected COVID-19 on images with an area under the curve (AUC) of 0.96.
The researchers then tested the model on an external validation dataset from Indiana University of 10,002 adult patients who tested positive and negative for COVID-19, with the model achieving an AUC of 0.72. On a second external validation dataset of 2,002 chest x-rays from Emory University, the model achieved an AUC 0.66.
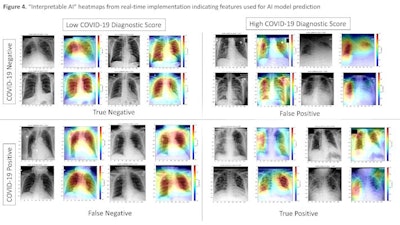
Next, in collaboration with Epic Cognitive Computing in Verona, WI, the researchers integrated the AI model into Epic at 12 University of Minnesota hospitals as a clinical decision-support system.
Between January and March 2021, the AI model evaluated all emergency department and inpatient chest x-rays in real-time of adult patients with a COVID-19 status of unknown or negative. During this period, there were chest x-rays from 5,077 participants that were negative for COVID-19 and 258 participants that were confirmed positive by RT-PCR.
According to results, participants with severe COVID-19 disease had higher scores versus participants with mild/moderate COVID-19 disease and participants without COVID-19, with a real-time AUC performance for the time period of 0.70
Lastly, an analysis revealed the COVID-19 AI diagnostic system had a significantly lower accuracy compared with two experienced radiologists, who had diagnostic accuracies of 67.8% and 68.6% compared to an AI model accuracy of 63.5%, the researchers found.
"AI-based diagnostic tools may serve as an adjunct, but not replacement, for clinical decision making of COVID-19 diagnosis, which largely hinges on exposure history, signs, and symptoms," the authors wrote.
The most significant challenge for AI models developed to diagnose COVID-19 on chest x-rays is that its radiographic appearance is heterogeneous, the researchers noted. The disease may range from no or minimal observable pathologic abnormality to severe acute respiratory distress syndrome, and it can progress and abate depending on the time of exposure and stage of disease.
Ultimately, at two years into the pandemic, the findings suggest that AI analysis of chest x-rays at best could be integrated by emergency department providers into clinical decision-making when developing a differential diagnosis and determining if patients need confirmatory testing and isolation for COVID-19, the authors wrote.
"As a future direction, we propose the integration of structured and unstructured data from electronic medical records into model training," they concluded.