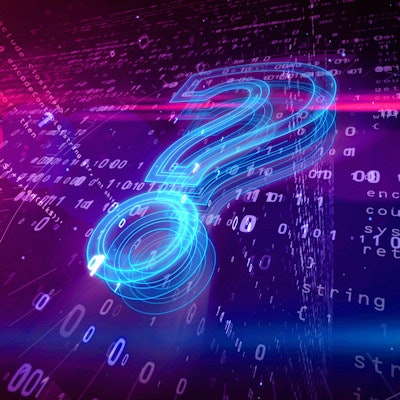
Despite an increase in regulatory approvals, not many artificial intelligence (AI) algorithms for medical imaging cleared by the U.S. Food and Drug Administration (FDA) reveal key information used in clinical studies, researchers have found.
This lack of transparency -- about patient demographics or machine specifications, for example -- could mean that those who use the algorithms aren't getting a clear picture of exactly what AI can do, and it could thus impede the adoption of potentially powerful tools, wrote a team led by Mihir Khunte from Brown University. The study results were published October 28 in Clinical Radiology.
"Although most [AI] devices utilize clinical data to support their use in real settings, very few expose the specific parameters of these studies, including patient demographics and machine specifications," Khunte and colleagues noted. "This crucial information can help consumers make an informed decision on whether the device is suitable for their practice."
The FDA has expanded its guidelines in recent years to reflect the changing landscape of AI's use in radiology. This includes classifying AI software into subgroups such as computer-aided detection, computer-aided diagnosis, computer-aided triage, computer-aided quantification, and image processing.
But although some research suggests that the market for AI in medical imaging will grow tenfold in the next decade, questions remain as to how it is validated.
The investigators explored the current state of FDA-approved AI products specifically designed for medical imaging, identifying trends in clinical validation strategy as reported in product FDA summaries. They conducted a study that included retrospective data from a total of 151 AI algorithms cleared by the FDA between 2008 and 2021. Out of these, algorithms using CT imaging were the most popular (49%), followed by MRI (25.2%).
They found that although 64.2% of the algorithm studies reported that clinical data were used to validate, only 4% revealed study participant demographics and 5.3% machine specifications. Just over a third (33.8%) of AI algorithms were characterized as having multicenter clinical data (key to producing robust results), while 2% had single-center data, and 64.2% did not specify. Ground truth used for clinical validation of the AI algorithm was specified in just over half (51.6%) of the FDA summaries.
Including more specific information about how AI algorithms are validated could help radiologists and their departments make better decisions about using AI, Khunte and colleagues wrote.
"As the intersection between AI and medical imaging continues to grow in importance, there is a need for greater transparency in how these devices are validated clinically, which will encourage both wider and safer adoption of these technologies into everyday practice," they concluded.