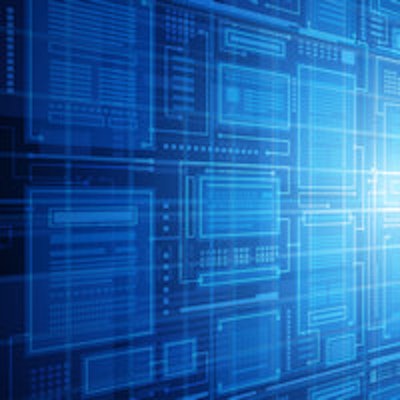
Big data and the software being developed to mine and learn from the vast amounts of data in clinical archives will pave the way for personalized cancer treatment, according to a presentation at the International Symposium on Multidetector-Row CT (MDCT) in San Francisco. And image data will be crucial.
By mining historical data in archives from patients with similar characteristics to a current patient and using that information to guide treatment decision-making, clinicians can truly pursue a model of evidence-based care, said Dr. Daniel Rubin, an assistant professor of radiology and medicine (biomedical informatics) at Stanford University.
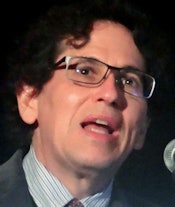
Development of these so-called rapid-learning systems so far has largely focused on patient survival statistics, however, and not made use of image information such as measurements and annotations. The latter are unstructured data that cannot be easily accessed and mined by computers, Rubin said. But as these image data become available in a structured format, rapid-learning systems will be able to tap into the crucial role imaging plays in assessing cancer treatment response.
"In the future, as [radiology participates] in this data realm of this ecosystem, we will add value," he said. "And we need to be central players in it, because we are in the 21st century and this paradigm is going to be important in terms of guiding decision-making."
Cancer is a heterogeneous disease, and radiologists need to help referring physicians and oncologists make the best treatment decisions for each patient based on the radiological findings, Rubin noted.
"The big problem they're concerned with and that [radiologists] need to be concerned with, and where big data can help, is understanding the notion of disease heterogeneity and which patients are going to respond better to particular treatments," he said. "Imaging, being important, needs to be a part of that if we're going to help guide oncologists to personalized treatments. Imaging is a crucial component of this evaluative process."
Currently, oncologists evaluate the imaging data and results, but their decision-making is largely based on published evidence or their own personal knowledge. This poses a number of challenges, however. It's difficult to use knowledge from the literature to guide individual decision-making, according to Rubin.
"All published evidence isn't applicable to all our patients; every patient is unique," he said. "Another problem is that there have been published articles that have shown that even the current evidence is insufficient to inform clinical decision-making because of its heterogeneity."
As a result, it can be difficult to match individual patients with the best evidence.
Rapid-learning systems
The development of rapid-learning systems for cancer would enable oncologists to find patients with similar characteristics to a given patient to determine treatments associated with a better outcome, such as survival or treatment response, according to Rubin. This could help alleviate the heterogeneity problem.
Imaging serves as the basis of treatment response; longitudinal imaging monitors how disease responds over time. Oncologists look at longitudinal image data as a graph of changes in disease burden over time with corresponding Response Evaluation Criteria in Solid Tumors (RECIST) labels.
"They also think about [image data] in terms of: What was the best response a patient had over time, and how did that cohort of patients do with that treatment?" he said. "What was their best response?"
However, these image data are difficult for electronic medical record software to extract because they are unstructured and not in a computer-accessible format, Rubin said. To solve this problem, initiatives are underway, such as the Annotation and Image Markup (AIM) standard developed by the National Cancer Institute's Cancer Imaging Program. AIM is a metadata standard that provides a syntax for recording measurements to make image content such as imaging observations, anatomy, region of interest, etc., mineable to computer applications.
Radiologists can then use tools such as ePad (developed by Stanford) to measure and annotate images in a format that can be parsed by a computer.
"Now we can bring the image data into the same computable, mineable space as the clinical data to enable this concept of rapid learning," Rubin said.
Killer app
The killer app for big data in medicine is rapid learning, according to Rubin. In addition to guiding treatment decisions now, rapid-learning will continue to help in the future, as current treatments become part of the medical record and can be used to inform decision-making for later patients.
"This rapid-learning paradigm is one of looking at historical evidence, helping them inform decision-making, making that decision, and then recording the outcomes," he said. "And that feeds back into future decision-making."
An example of such a rapid-learning system was developed by Stanford and Vanderbilt-Ingram Cancer Center. Patient data including disease, demographics, treatment, genetic profile, image measurements, and outcomes from 260 melanoma patients were entered into a centralized database. This database can then be mined to construct patient cohorts based on a range of variables, Rubin said.
It can also filter cohorts further to control for sex, age, genetic results, drug class, and drug name. Cohort statistics can then be generated on the fly, according to Rubin.
"As we get our imaging results out of this arcane text format into [a format that can be processed by computers], we can get our image data participating in an emerging big-data paradigm of discovery, where physicians -- instead of just relying on what they have in their head and what they read in the literature -- can access this virtual database of mineable data in hospital enterprises or across enterprises to guide their decision-making," he said.