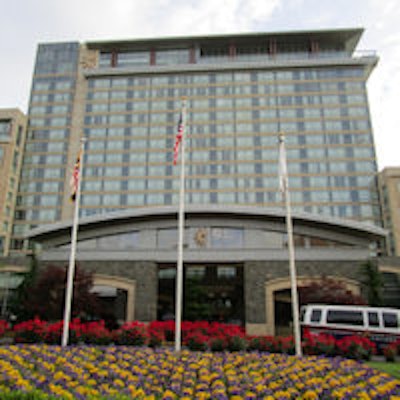
NATIONAL HARBOR, MD - A new data-mining algorithm goes beyond the capabilities of most software applications to unearth subtle imaging findings in vast PACS databases, and could be beneficial for research applications, according to a presentation on Thursday at the Society for Imaging Informatics in Medicine (SIIM) 2015 annual meeting.
Sorting through a virtual haystack of more than 9 million datasets in the vast PACS network at Henry Ford Hospital in Detroit, the group's Data Fish Pro algorithm found the needles it was looking for -- that is, knee images with evidence of post-traumatic bone marrow edema at MRI based on imaging features.
"In the context of radiology, it gets around a lot of the issues of nonstandardized reporting with nonstandard keyword searches that maybe aren't in the reports at all," said lead investigator Dr. Brendan Kelley, a radiology resident at Henry Ford Hospital. The potential for new research based on subtle imaging findings is potentially huge, he added.
Better data mining needed
Searching through databases for specific imaging findings for research purposes is tedious work, requiring time and manual labor. Without standardized reporting there are many different ways findings are reported, and current software is very limited in its ability to collect specific data, Kelley said.
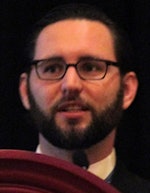
For example, current techniques are limited in their ability to find images with mutually inclusive variables, and complex searches often lead to incomplete results that do not identify all patients with certain radiologic findings.
"You can't really look for specific imaging findings seen in different imaging modalities at certain periods of time," Kelley said.
The software on which the algorithm was built "is kind of a hybrid between data mining and specific software," he said.
The Data Fish technique works in three phases: First, data are imported with specific imaging criteria, and then a customized algorithm creates a master list of patients. Finally, researchers take over the task, doing a manual data sort to finalize the list of patients.
For this project, Kelley and colleagues performed a retrospective review of PACS data to learn the significance of bone marrow edema present after acute knee trauma but absent acute fractures, advanced arthritis, or anterior cruciate ligament (ACL) tears.
The patients needed to have an MRI and an x-ray of the knee within a month, at least one follow-up x-ray, and bone marrow-related signal changes on MRI. The dataset included approximately 400,000 knee x-rays and 26,000 knee MRI scans. About 700 patients met all of the criteria.
The graphical user interface is designed to display lists of MRI and x-ray findings side by side, which allowed Kelley to narrow the list quickly to about 50 patients who would be ideal for the group's research, he said.
Broad potential applications
The two-week data-mining project was very successful, he said.
"We developed a format to identify a highly specialized patient population," Kelley said. "It's hard to say if this would have been possible with manual sorting techniques. It definitely would have taken a lot longer."
The group has already started applying the algorithm to other research projects, with continuous updates and improvements. The user interface allows for quick inclusion and exclusion of datasets, and cases can be flagged for additional chart review.
"Many of the challenges we face when we try to do large retrospective studies will be minimized when we move to standardized reporting, but in the meantime, we have all this data and we should be using it," Kelley said.
Moving forward, there are many improvements to make, but the biggest ones are establishing the software as an independent platform, eliminating the need to "recode" the algorithm for each project, and making the software able to incorporate other types of data, such as from electronic medical records, he said.