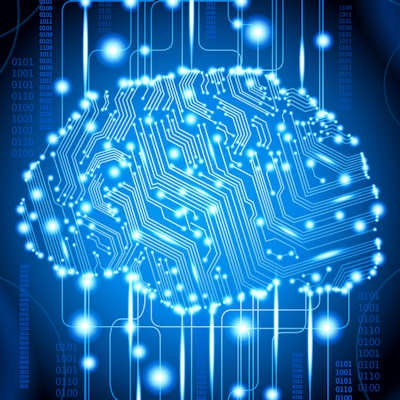
The combination of artificial intelligence (AI) and radiomics features on CT images can outperform current methods for preoperatively predicting lymph node metastasis from gastric cancer, according to research published online February 4 in the Journal of the American College of Radiology.
Researchers from China developed a machine learning-based software application to automatically predict lymph node metastasis from the analysis of CT radiomics features. The software was more accurate than radiologist predictions of metastasis based on a conventional staging criterion, they found.
"A [decision-support system] based on 13 'worrisome' radiomics features appears to be a promising tool for the preoperative prediction of [lymph node] status in patients with [gastric cancer]," wrote the researchers led by Dr. Qiu-Xia Feng from the First Affiliated Hospital of Nanjing Medical University.
It's important to have accurate preoperative evaluation of lymph node status when determining an appropriate treatment strategy for gastric cancer and predicting prognosis. However, it can be difficult to distinguish malignant lymph nodes on CT and MRI, especially when the nodes are small or contain micrometastases, according to the researchers.
"Hence, modalities for accurate preoperative detection of [lymph node] metastasis are urgently needed," they wrote.
Feng and colleagues sought to develop a machine learning-based automatic clinical decision-support system that could provide preoperative analysis of the risk for lymph node metastasis in these patients by analyzing clinical and imaging data. After retrospectively collecting clinicopathologic and CT imaging data from 490 patients diagnosed with gastric cancer between January 2002 and December 2016, the researchers extracted radiomics features from venous-phase CT images. Of the 490 patients, 297 (60.6%) had histopathologic evidence of lymph node metastasis.
The researchers selected, ranked, and modeled the relevant features using a support vector machine classifier on 326 training and validation datasets. The resulting model yielded an area under the curve (AUC) of 0.804 in the testing and validation dataset and an AUC of 0.764 in the remaining 160 test cases. The group also compared the diagnostic performance of the software with a conventional staging criterion, as assessed on CT images by two radiologists with three years and 10 years of abdominal imaging experience, respectively. The radiologists independently provided their evaluations and were blinded to the clinical and pathological data.
In the radiologist evaluations, the threshold for a metastatic lymph node was set at a short-axis node size of larger than 10 mm. If one or more lymph node metastases were discernible in the group, then the nodes were considered to be positive. The radiologists resolved any discrepancies in the evaluation via consensus.
Accuracy of AI + radiomics for predicting lymph node metastasis | ||
Evaluation using conventional staging criterion | Machine-learning algorithm | |
Training and validation data | 63.5% | 76.4% |
Testing data | 63.2% | 71.3% |
"Therefore, the predictive power of our model is obviously superior to that of conventional contrast-enhanced CT, which is presently widely used for [lymph node] staging in clinical practice," the researchers wrote.
The method now awaits external, multicenter, evidence-based validation, they concluded.