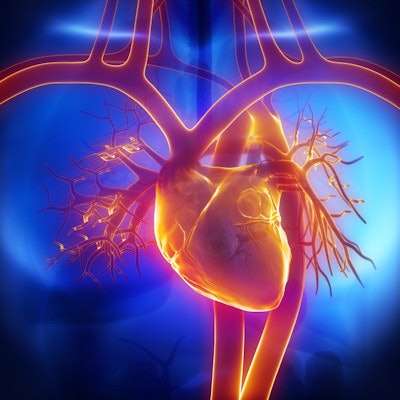
The combination of plaque quantified on coronary CT angiography (CCTA) and artificial intelligence (AI)-powered fractional flow reserve (FFR-CT) analysis can accurately predict future major adverse cardiac events, according to research published online August 8 in the American Journal of Cardiology.
Using a prototype deep-learning software application, a multinational research team led by Dr. Philipp von Knebel Doeberitz of the Medical University of South Carolina and Mannheim-Heidelberg University in Mannheim, Germany, found that FFR-CT and plaque markers derived on CCTA could identify patients who would have a future major adverse cardiac event significantly better than just CCTA stenosis grading alone.
CCTA-derived plaque markers and FFR-CT based on machine learning demonstrate value in identifying major adverse cardiac events, the authors wrote.
Stenosis 50% or more and several plaque features measured on CCTA have been shown in the literature to provide prognostic power for these cardiac events, while deep learning-based FFR-CT also has been validated for identifying lesion-specific ischemia, according to the researchers. To evaluate the potential effect of a combination of the two methods, they analyzed 82 patients who had received CCTA and invasive coronary angiography for known or suspected coronary artery disease (CAD) at a single center between October 2012 and July 2017. Of the 82 patients, 18 (21%) developed a major adverse cardiac event during the study's median follow-up period of 18.5 months.
Next, the researchers retrospectively utilized prototype software from Siemens Healthineers to quantify plaque for control lesions and the lesions responsible for the cardiac event; another prototype using a deep learning-based software application from Siemens was used to perform FFR-CT analysis.
After conducting multivariate analysis, the researchers found that five markers -- lesion length, low-attenuation plaque, napkin ring sign, stenosis of 50% or more, and FFR-CT of 80% or less -- were significant predictors for major adverse cardiac events. They then compared the performance of three different decision-making strategies for predicting these events and found that a combination of markers worked the best.
Performance of markers for predicting major adverse cardiac events | |||
Stenosis ≥ 50% | Combination of stenosis ≥ 50% and plaque markers | Combination of stenosis ≥ 50%, plaque markers, and FFR-CT ≤ 80% | |
Area under the curve | 0.60 | 0.87 | 0.94 |
"The combined approach of anatomical stenosis grading with the addition of plaque characteristics showed superior value over stenosis assessment alone," the study authors wrote. "However, the addition of [FFR-CT] analysis to visual stenosis grading on [CCTA] and plaque quantification resulted in incremental predictive value."
Coronary plaque quantification yields information that may further inform treatment decision-making and enable sufficient risk stratification and subsequent lifestyle modifications, according to the researchers.
"Although there is robust data on the value of plaque quantification and characterization for sufficient risk stratification beyond stenosis grading on [CCTA] alone, the manual or semiautomated plaque analysis is rather time-consuming and requires manual adjustment," the authors wrote. "Thus, fully automated or machine learning-based applications incorporating big data derived from [CCTA] will most likely guide the future of cardiac imaging. In addition, with [FFR-CT], this combined anatomical and morphological information will provide more insight into clinical decision-making of CAD and outcome prediction."