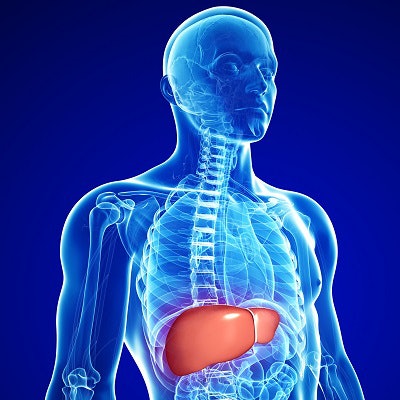
An artificial intelligence (AI) algorithm was able to automatically quantify liver fat on abdominal CT images in a new study, according to research published online September 17 in Radiology. The findings may potentially enable patients who are undergoing CT scans for other indications to also be screened for nonalcoholic fatty liver disease (NAFLD).
After testing on nearly 11,700 nonenhanced CT studies, a team of researchers from the University of Wisconsin School of Medicine and Public Health in Madison and the U.S. National Institutes of Health (NIH) Clinical Center in Bethesda, MD, found that their deep-learning algorithm was highly accurate for assessing hepatic steatosis.
"This fully automated CT-based liver fat quantification tool allows for population-based assessment of hepatic steatosis and nonalcoholic fatty liver disease, with objective data that match well with manual measurement," wrote leader author Peter Graffy and colleagues.
Although MRI with a proton density fat fraction sequence has emerged as the noninvasive method of choice for quantifying liver fat, liver fat measurements on nonenhanced CT have also been shown to correlate well with that technique. As abdominal CT is performed more frequently than MRI for various indications in clinical practice, this could potentially allow those exams to also be utilized for population-based screening for hepatic steatosis and nonalcoholic fatty liver disease, according to the authors.
Having recently trained and tested a deep-learning algorithm for performing CT-based liver segmentation and attenuation assessment, the researchers sought to apply this model for assessing the presence of hepatic steatosis in a large asymptomatic adult screening cohort. The liver segmentation algorithm employs 3D convolutional neural networks to perform automated volume-based liver attenuation measurements, including conversion to CT fat fraction.
The researchers assessed the algorithm's performance on 11,669 CT scans from 9,552 adults -- 5,314 women and 4,238 men -- with a mean age of 57.2 years. The studies also included 2,117 follow-up scans with a mean interval of 5.5 years in 1,862 adults. The automated volume-based liver attenuation measurements had a mean difference of 2.7 Hounsfield units (HU) with manual measurements -- yielding a correlation (r2) of 0.92.
The AI algorithm also identified hepatic steatosis in more than half of the screening cohort, the researchers noted. In addition, 10% of the cohort had moderate or severe steatosis.
Delving further into the data, the team also found only a weak correlation of hepatic steatosis with body mass index, age, and sex. This indicates that clinical demographic and morphometric assessment can't effectively predict the likelihood of underlying nonalcoholic fatty liver disease, according to the authors.
In other findings, the researchers noted a mean change of only -3 HU between the first CT scan and the follow-up study. However, 806 (43.3%) of the 1,861 patients had a change in their steatosis category between the two exams.
"If hepatic steatosis is shown to be an independent risk factor for future adverse events, then this automated tool could also be potentially used for opportunistic NAFLD screening with any nonenhanced CT that includes the liver (abdominal or thoracic), regardless of the clinical indication for imaging," the authors wrote.