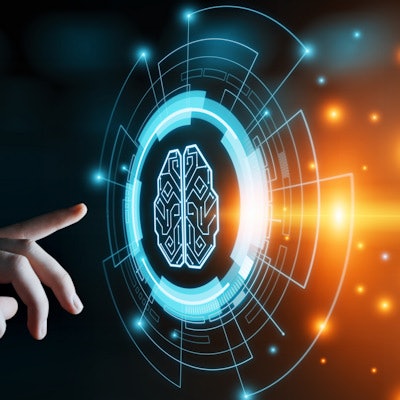
A deep-learning algorithm can help chest CT better quantify lung opacification caused by COVID-19, according to a study by researchers from Wuhan, China, published March 30 in Radiology: Cardiothoracic Imaging.
The findings suggest a way to reduce subjectivity in radiologists' initial assessment and follow-up of COVID-19 pulmonary findings on CT, wrote a team led by Dr. Lu Huang, PhD, of Huazhong University of Science and Technology in Wuhan.
"Quantitative analysis of the CT scans using an artificial intelligence tool, in particular deep learning, could provide an automatic and objective estimation of the disease burden, facilitating and expediting imaging interpretation during the COVID-19 pandemic," the group wrote.
Radiologists evaluate chest CT scans using visual scoring, but scores can vary among readers. Huang's group sought to explore whether a deep-learning algorithm could mitigate this variation and thus make both baseline and follow-up chest CT scans for COVID-19 more effective. The algorithm featured a lung and lobes segmentation module, a pneumonia segmentation module, and a quantitative analysis module.
The study included 126 patients categorized into those with mild, moderate, severe, and critical disease who underwent chest CT between January and February. The deep-learning software evaluated the opacification of the whole lung and five lobes, comparing initial CT scans with follow-up exams.
These results were then compared with the assessment of two radiologists, who reviewed the deep-learning results as regions of interest overlaid on the CT images. The researchers used a scale of 0 to 5 to compare agreement between the deep-learning algorithm and the radiologists, with a score of 3 or higher considered sufficient agreement; more than 91% of the algorithm's lung opacity assessments met this score.
Of the 126 patients, six had mild disease, 94 had moderate, 20 had severe, and six were critical, according to Huang and colleagues. Lung opacity increased on a statistically significant basis for all three groups when the team compared baseline CT exams with the first follow-up scan: The deep-learning algorithm's whole-lung opacification percentage increased from 3.6% at baseline to 8.7% at first follow-up (p < 0.01).
The study findings suggest that deep learning could help clinicians better evaluate the severity of the pulmonary manifestations of COVID-19, according to the researchers.
"There were significant differences in lung opacification percentage, as measured by the deep-learning algorithm, among patients with different clinical severity," the team wrote. "This automated tool for quantification of lung involvement may be used to monitor the disease progression and understand the temporal evolution of COVID-19."