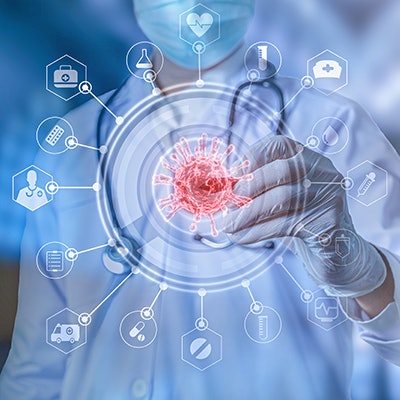
Machine learning-based analysis of chest CT reports for respiratory illness findings could provide advance warning of new COVID-19 hot spots, according to a study published online February 25 in Radiology: Cardiothoracic Imaging.
Researchers led by Dr. Ricardo Cury of Mednax Radiology Solutions applied natural-language processing (NLP) algorithms to search over 400,000 chest CT reports from their national radiology group for findings associated with respiratory illness. Forecast models based on these NLP results yielded predictions of new COVID-19 cases that correlated highly on a daily, weekly, and state-by-state basis with the number of official cases announced later.
"This nationwide surveillance algorithm has the potential to help health care entities and public health authorities develop strategies against COVID-19 and other similar pandemics in the future," the authors wrote.
The researchers set out to determine if an NLP algorithm could extract keywords from chest CT imaging reports in order to generate predictions. They also sought to compare these predictions with officially recorded COVID-19 cases and deaths in the U.S.
Three different NLP algorithms were developed. One, called Viral Pneumonia NLP, was designed to search chest CT reports for keywords related to viral pneumonia. The second, called Imaging Findings NLP, searched for typical imaging findings found in COVID-19 patients. The last NLP model, called COVID NLP, searched for specific words that indicated viral pneumonia and COVID-19.
The researchers also utilized two forecasting models from Microsoft to prospectively predict new COVID-19 cases based on the NLP analysis. The first model, which is based on an artificial neural network, provides predictions for new cases from analysis of NLP results and historical data. The second model creates long-term forecasts by employing forecasting methodology to model time series data with complex seasonal patterns, according to the researchers.
They applied the three different NLP algorithms to 450,114 patient chest CT reports that were produced between January 1 and October 3, 2020, at over 2,100 facilities in 50 states. The best performing algorithm -- COVID-19 NLP -- yielded strong correlation (r2 = 0.82) with daily COVID-19 cases.
The NLP models showed that increases in official cases were preceded by an early rise of cases with respiratory illness findings, suggesting the possibility that these findings could serve as an early predictive marker for official cases on a weekly basis (r2 = 0.91), according to the researchers. Respiratory illness findings also correlated substantially with official COVID-19 incidence per U.S. state.
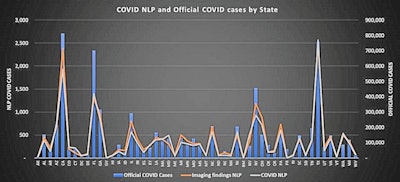
"It is important to note that the early rise of cases detected by both NLP models occurred before the rise of new official COVID-19 cases during the first wave," the authors wrote.
In discussing the need for this capability, the researchers acknowledged that Johns Hopkins University's COVID-19 Data Repository is a credible source of data for new COVID-19 cases and death. The U.S. Centers for Disease Control and Prevention (CDC) also provides U.S. COVID-19 data on a city level.
"Nevertheless, the utility of an early warning system using chest CT findings may in fact shine in the absence of laboratory data, as chest CT abnormalities can identify a regional spike in respiratory illness before a virus has even been isolated or, if already isolated, if viral testing is not yet widely available," the authors wrote.