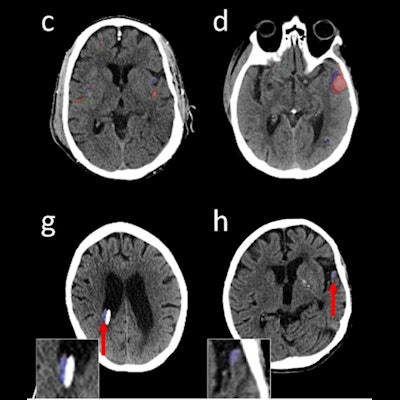
Using an artificial intelligence (AI) algorithm with noncontrast head CT scans helps clinicians better identify, localize, and characterize intracranial hemorrhages, according to a study published April 20 in Radiology: Artificial Intelligence.
The findings suggest that using AI to analyze brain hemorrhages could not only improve patient care but streamline radiology department workflow, said a team led by Eli Gibson, PhD, of Siemens Healthineers in a statement released by the RSNA.
"The hope is that AI systems for diagnostic imaging could help reduce errors of perception and/or interpretation and thus reduce uncertainty," the group said.
Noncontrast CT is commonly used to assess urgent neurologic conditions such as stroke and head trauma, the team noted. It's especially effective for identifying acute intracranial hemorrhage, which has a one-month mortality rate of 40% -- making it imperative to diagnose it accurately and quickly. AI could help minimize time to interpretation and time to treatment.
"AI algorithms triggered immediately after image acquisition could potentially flag hemorrhages to inform image interpretation and therapy protocols, to notify radiologists or other clinicians, and to reprioritize radiology worklists," the authors wrote.
But AI algorithms aren't perfect, a fact that can affect the trust clinicians are willing to place in them. In a study supported by Siemens, Gibson's group evaluated the performance of an AI method that identifies, types, and locates acute and subacute intracranial hemorrhage on noncontrast CT exams and generates a detection confidence score that could improve interpretation accuracy and radiology worklist prioritization.
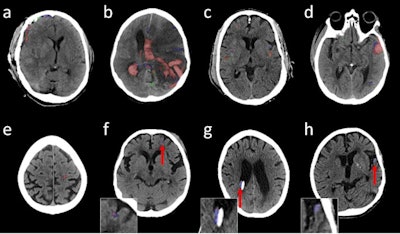
The research included 46,057 CT studies; data was categorized as "internal" (for training of the model) and "external" (for calibration and evaluation of it). The algorithm's performance was measured using area under the receiver operator characteristic curve (AUC ROC) analysis and report turnaround time. The authors also estimated two confidence scores (calibrated classifier [CC] and Dempster-Shafer [DS], both based on a scale of 0 to 1, with higher scores denoting higher confidence).
AUC ROC measures were high -- particularly with the internal, or training, dataset -- demonstrating good performance from the algorithm in detecting, localizing, and subtyping intracranial hemorrhage.
Performance of AI algorithm for hemorrhage detection and subtyping of CT exams | |||
Type of hemorrhage | AUC | Sensitivity | Specificity |
Internal (training) set | |||
Intracranial | 0.97 | 92% | 93% |
Subarachnoid | 0.90 | 87% | 82% |
Subdural | 0.92 | 84% | 89% |
Epidural | 0.93 | 90% | 78% |
Intraparenchymal | 0.92 | 90% | 84% |
Intraventricular | 0.96 | 89% | 93% |
External (calibrating/evaluating) set | |||
Intracranial | 0.95 | 86% | 92% |
Subarachnoid | 0.88 | 84% | 78% |
Subdural | 0.85 | 76% | 83% |
Epidural | 0.77 | 56% | 83% |
Intraparenchymal | 0.92 | 88% | 84% |
Intraventricular | 0.96 | 90% | 92% |
The CC confidence score was 0.93 and the DS score was 0.92 for the internal dataset and 0.88 and 0.89 for the external dataset. The group also found that the AI algorithm showed potential to help reduce report turnaround time by 25% to 27%.
Using the AI algorithm with head CT could translate into better patient care, the team noted.
"Identifying hemorrhage subtypes and segmentation with automated measurement of hemorrhage location and volume could allow for more precise prioritization," it concluded.