Adding thoracic aortic calcification (TAC) data to coronary artery calcification (CAC) AI models shows promise as a risk prediction biomarker for CAC in asymptomatic patients undergoing chest CT, researchers have reported.
The findings could help clinicians better understand the possible prognostic function of TAC, according to a team led by Young Joo Suh, MD, PhD, of Yonsei University College of Medicine in Seoul, South Korea. The results were published March 26 in the American Journal of Roentgenology.
"AI-based quantification provides a standardized approach for better understanding the potential role of TAC as a predictive imaging biomarker," Suh said in a statement released by the AJR.
Increased use of chest CT for lung cancer screening has also increased incidental detection of calcifications in the heart, including thoracic aorta calcifications, the group explained. Previous research has noted the potential clinical significance of incidental TAC found on routine CT imaging in a general screening setting, finding a higher risk of major adverse cardiovascular events (MACE) and all-cause mortality (ACM) to be associated with TAC and CAC. But the importance of using TAC and CAC data to predict these events has remained uncertain.
To attempt to clarify the question, Suh and colleagues investigated any long-term prognostic implications of TAC assessed using AI-based quantification on routine chest CT imaging in a screening population. Their study included 7,404 asymptomatic individuals who underwent nongated, noncontrast chest CT as part of a national general health screening program between January 2007 and December 2014 (of these, 79.3% were men, and 64.8% were ever-smokers; 22.5% had a history of hypertension).
The group employed a commercial AI program (ClariCardio from ClariPi) that used the Agatston method to quantify segmented TAC and CAC; the team then stratified these scores into categories. Two radiologists manually quantified TAC and CAC for 2,567 examinations. The researchers assessed the role of AI-based TAC categories for predicting MACE and ACM -- independent of AI-based CAC categories as well as clinical and laboratory variables, they noted.
The researchers found the following:
- In Kaplan-Meier analyses, both AI-based TAC and CAC categories were significantly associated with both MACE and ACM.
- In 2,567 CT exams in which both AI-based and manual quantification of TAC and CAC were conducted, TAC and CAC showed "excellent" agreement (concordance correlation coefficient, or CCC, of 0.97 and 0.9, respectively, with 1 as reference).
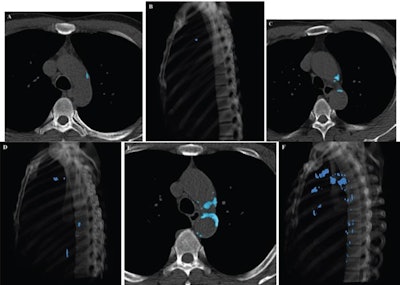
The takeaway? It's possible that AI-based TAC data used with routine chest CT imaging could improve assessment of patients' heart disease risk.
"[We found that] AI-based quantification was useful to help address ongoing controversy regarding the utility of TAC, independent from CAC, as a prognostic marker," the researchers concluded.
The complete study can be found here.