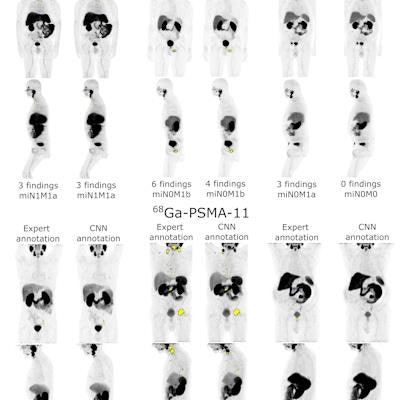
An AI model based on prostate-specific membrane antigen (PSMA)-PET/CT imaging performs well in staging prostate cancer patients for surgery, according to a talk at the Society for Nuclear Medicine and Molecular Imaging (SNMMI) meeting in Chicago.
Nicolo Capobianco, PhD, a research scientist at Siemens Healthineers in Erlangen, Germany, and colleagues at the Lawson Health Research Institute in London, Ontario, developed a model that identifies regions suspicious for cancer and that agreed with a physician expert.
"The proposed method is promising to support prostate cancer staging based on PSMA-ligand PET/CT," Capobianco told attendees in a June 24 session on basic and translational oncology research.
In recent years, researchers have developed PET radiotracers that reveal prostate cancer based on their ability to bind to overexpressed PSMA protein in primary tumors, as well as cancer that has spread to lymph nodes, bone, and other sites. Previous studies have shown that PSMA-PET/CT is highly accurate and can ultimately predict survival outcomes in patients.
Yet interpreting radiotracer uptake, especially in small metastatic lesions, requires careful and time-consuming visual assessments of images by trained nuclear medicine physicians, Capobianco explained. With these physicians in high demand, the group hypothesized that AI may help.
In the study, the authors developed and tested a convolutional neural network (CNN)-based method to classify cases based on a standardized molecular imaging framework developed several years ago for staging tumors and nodal and metastatic cancer (miTNM, version 1.0). miTNM is designed to organize findings in comprehensible categories for local (T), pelvic-nodal (N), and metastatic (M) disease to promote the exchange of information among physicians and institutions.
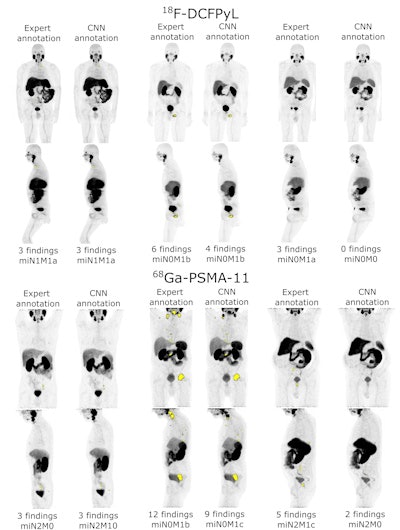
The dataset included 173 prostate cancer images of patients who underwent PET/CT using F-18 DCFPyL radiotracer (Pylarify) and 193 patients who underwent PET/CT using gallium-68 (Ga-68) PSMA radiotracer. Both PET tracers are approved in the U.S. In training, the group ultimately pooled data for both tracers, Capobianco noted.
"Training a single nnU-Net network for both radiotracers resulted in overall higher performance for detecting suspicious sites compared to training separate networks for each radiotracer," he said.
In testing, the researchers compared the model's performance to that of an expert who identified 1,207 suspicious findings on the PSMA-PET/CT images and who classified the cancer based on the miTNM system.
The AI model matched the expert's assessment of local and nodal cancer in 65% of cases that used F-18 DCFPyL and 79% of cases for Ga-68 PSMA-11; for metastatic lesions, the AI model matched the expert's assessment in 73% of cases that used F-18 DCFPyL and 75% cases that used Ga-68 PSMA-11, according to the findings.
"Subjects imaged with Ga-68 PSMA-11 had higher disease burden, potentially underlying these observed differences in staging performance," Capobianco noted.
Ultimately, deep-learning methods have shown promising results for automatically identifying sites suspicious for cancer and have potential in prostate cancer staging using PSMA-ligand PET/CT imaging, with this study providing further support for the approach, Capobianco concluded.
"Deep-learning methods can identify regions suspicious for cancer and determine the miTNM nodal and metastatic stage in F-18 DCFPyL and Ga-68 PSMA-11 PET/CT images with moderate to good agreement with an expert physician," he said.