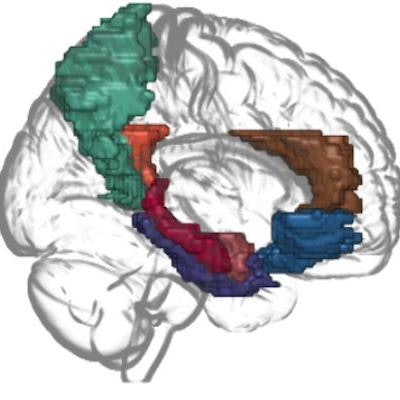
Using functional MRI (fMRI), a research team from the University of Texas at Austin has developed what it calls a "noninvasive language decoder" that could potentially offer a window into the mind of a patient who's incapable of speaking.
The decoder, which is designed to "reconstruct the meaning of perceived or imagined speech," could help people suffering from conditions like locked-in syndrome or Lou Gehrig's disease (i.e., amyotrophic lateral sclerosis, or ALS), according to a group led by doctoral candidate Jerry Tang. The study findings were published May 1 in Nature Neuroscience.
"The goal of language decoding is to take recordings of brain activity and predict words a user is hearing or ... imagining," Tang said in a press conference held May 1. "[It could be good news for individuals who have] lost the ability to speak due to injuries or diseases."
Speech decoder technology already exists, and it has been applied to neural activity after invasive neurosurgery to implant devices intended to help individuals communicate, Tang and colleagues noted. There are also noninvasive decoders that use brain activity recordings, although these have been limited to single words or short phrases, making it "unclear whether these decoders could work with continuous, natural language," the authors explained.
In any case, decoder technology is generating media buzz: most recently, a March article in Scientific American described how, although image-generating artificial intelligence technology is "getting better at re-creating what people are looking at from ... fMRI data ... it's not mind reading -- yet."
In their study, Tang and senior author Dr. Alexander Huth and colleagues sought to address the knowledge gap regarding noninvasive decoder technology's ability to reconstruct continuous language output via a study that used fMRI to gather recordings from three participants exposed to 16 hours of content (stories from The Moth Radio Hour and episodes of the New York Times' Modern Love podcast and "silent" Pixar shorts [i.e., no dialogue]); this phase of the research was used to train the decoder. The investigators then had participants listen to new stories and tested the decoder's ability to generate word sequences that captured the meanings of the new content.
The study found that the decoder could "predict the meaning of a participant's imagined story or the contents of a viewed silent movie from fMRI data," according to a statement released by the university, and that "when a participant actively listened to a story ... the decoder could identify the meaning of the story that was being actively listened to."
"We found the decoder ... could recover the gist of what the user heard," Tang said in the press conference. "[For example, when the user heard] 'I don't have my driver's license yet,' the decoder predicted 'She has not even started to learn how to drive yet.' "
The team also performed a "privacy analysis" for the decoder, discovering that when it was trained on one participant's fMRI data, its ability to predict responses from another individual was limited.
"You can't train a decoder on one person and then run it on another person," Tang said.
Finally, the group tested whether the decoder would work without subject cooperation, noting that "nobody's brain should be decoded without permission," and whether it could be undermined by study subjects through counting, listing animals, or telling themselves a different story silently in their heads.
"Especially naming animals and [alternative stories] sabotaged the decoder," Tang said.
The study underscores the importance of careful research on the privacy implications of decoder technology, according to Tang.
"While this technology is in its infancy it's important to regulate what it can and can't be used for," he cautioned. "If it can eventually be used without a person's permission, there will have to be [stringent] regulatory processes, as a prediction framework could have negative consequences if misused."
The group has made its custom decoding code available at github.com/HuthLab/semantic-decoding.
Disclosure: Huth and Tang have submitted a patent application under the auspices of the University of Texas System directly connected to this research.