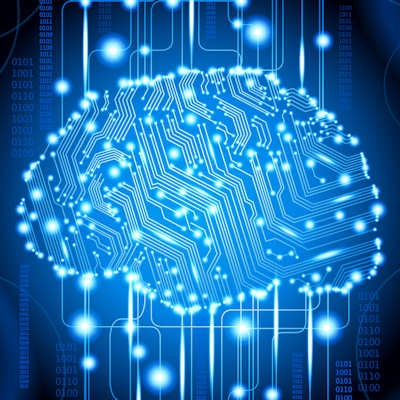
Can a computer-aided diagnosis (CADx) system based on machine learning characterize focal liver lesions on contrast-enhanced ultrasound (CEUS) cine clips as accurately as an expert reader? It's certainly possible, if research published online recently in Radiology is any indication.
A multi-institutional team found that not only was the internally developed CADx algorithm significantly better than an inexperienced radiologist for differentiating these cases, but it also performed comparably to a radiologist who had more than two decades of experience with CEUS. What's more, the system showed promise as a tool for improving the accuracy of both radiologists.
"[CADx] systems that accurately analyze contrast-enhanced US cine clips to distinguish benign from malignant [focal liver lesions] can be integrated into the diagnostic workflow to confirm observer diagnoses or to flag lesions for further review to improve overall diagnostic accuracy," wrote the team led by Casey Ta, PhD, from the University of California, San Diego (UCSD).
Experience counts
Although contrast has been shown to improve the performance of ultrasound for detecting and characterizing focal liver lesions, reliable and accurate characterization of tumors requires experienced radiologists due to the destruction of contrast microbubbles and variations in tumor behavior and enhancement. However, there are only a limited number of radiologists who are experienced in performing and interpreting CEUS studies, and interobserver variability continues to be an issue, according to the researchers (Radiology, October 25, 2017).
CADx could potentially help solve these problems. Current CADx systems typically extract B-mode features and/or CEUS videos; machine-learning algorithms are then trained to associate these features with the known diagnoses in order to predict the diagnoses of unknown lesions, according to the researchers. These CAD systems have generally captured time-intensity curves and calculated properties such as peak enhancement, time to peak enhancement, and area under the curve; other features have also been considered, including relative enhancement between the lesion and the parenchyma, lesion rim versus lesion center, enhancement homogeneity, lesion morphology, and vessel morphology. These approaches have only been evaluated on a limited number of lesion types, however.
Ta and colleagues noted, though, that human readers utilize hallmark B-mode appearances and enhancement patterns to detect and characterize focal liver lesions. As a result, it would seem that these patterns may also enable CADx software to accurately classify these cases, according to the researchers. To explore this possibility, they performed a retrospective study to assess the performance of an internally developed CADx system. They also wanted to determine the features on CEUS cine clips that were most useful for characterizing these focal liver lesions.
Assessing CADx
The researchers gathered 105 three-minute contrast ultrasound cine clips of focal liver lesions from subjects at three independent sites -- UCSD, the University of Alabama at Birmingham, and Southwoods Imaging in Youngstown, OH -- that had participated in a multicenter clinical trial of the SonoVue ultrasound contrast agent (Bracco Diagnostics). Of the 105 cases, 54 were malignant and 51 were benign.
Next, they used custom software developed using the Matlab R2015a computing environment (MathWorks) to perform in-plane motion correction and out-of-plane motion filtering on the images. This enabled pixel-by-pixel analysis, according to the group. Two freehand regions of interests (ROIs) were manually drawn on the B-mode images, one circumscribing the focal liver lesion and the other around normal liver parenchyma.
A total of 92 B-mode morphological image features, contrast enhancement features, and pixel time intensity curve (TIC) features were then analyzed using two different machine-learning classifiers: a support vector machine (SVM) and an artificial neural network (ANN). The researchers assessed the performance of both classifiers using 10-fold cross-validation testing.
To assess the potential clinical use of CADx, the researchers also compared its performance with that of two readers, including a radiologist who has more than 20 years of experience in performing and interpreting CEUS. The second radiologist had no prior CEUS experience but had attended a one-hour workshop on interpreting CEUS images.
The CADx system was able to analyze 95 of the 105 cine clips; 10 could not be assessed due to reasons such as excessive motion, absence of normal liver parenchymal enhancement, or poor contrast enhancement. The inexperienced reader was able to classify 100 cine clips; five couldn't be analyzed due to poor enhancement or the lesion being too small to characterize. Meanwhile, the experienced reader classified 102 cine clips; poor image quality or poor enhancement prevented assessment of the other three cases.
Comparable performance
The SVM algorithm outperformed the inexperienced reader and performed comparably to the experienced reader for differentiating benign and malignant focal liver lesions:
- Support vector machine (SVM): area under the curve (AUC) = 0.883
- Artificial neural network (ANN): AUC = 0.829
- Experienced reader: AUC = 0.843
- Inexperienced reader: AUC = 0.702
The difference in AUC between the inexperienced reader and the SVM was statistically significant.
"[CADx] systems accurately classified 11 different [focal liver lesion] types as benign or malignant by analyzing three-minute contrast-enhanced US cine clips acquired as a dual display to automatically extract hallmark sonographic, enhancement, and TIC features," the group wrote.
The researchers noted that the CADx system using the SVM was accurate in 77 (81.1%) of 95 cases.
"Equally important, when [CADx] classification was in agreement with that of an experienced or inexperienced reader, it increased their accuracy to nearly 90%," they wrote.
Radiologist accuracy for characterizing focal liver lesions | ||
Reader experience | Overall accuracy | Accuracy when interpretation agreed with CADx classification |
Inexperienced | 67/94 (71.3%) | 57/65 (87.7%) |
Experienced | 76/94 (80.9%) | 65/72 (90.3%) |
When the CADx algorithm disagreed with the inexperienced reader, the experienced reader's classification was used as the tiebreaker for the purpose of the study. Adding the experienced reader's opinion for these cases improved accuracy from 34.5% (10/29 cases) to 82.8% (24/29 cases).
"[CADx] systems could provide a second opinion to improve confidence when classifications agree or to flag ambiguous lesions for additional review when they disagree," they wrote.
The researchers also found that B-mode heterogeneity and contrast material washout were the most discriminating features selected by CADx for all iterations. In 207 (99%) of 209 cases, the CADx algorithms selected TIC features to classify the focal liver lesions; intensity-based features were used in two (1%) of the 209 cases.
In addition, none of the 15 criteria used to assess video quality were found to have a statistically significant effect on the accuracy of the CADx algorithms.
Limitations
The researchers acknowledged a number of limitations to the study, including its small sample size and a limited number of lesions that were smaller than 2 cm. The system also did not account for features of liver background, which could affect performance.
"The system developed here classified [focal liver lesions] solely on the basis of [CEUS] imaging data; however, we anticipate that the inclusion of pretest probability factors (e.g., Child-Pugh score, serum α-fetoprotein levels, CT or MR imaging findings, history of prior malignancy) will improve diagnostic accuracy," they wrote.
The system took an average of 64.5 minutes per study to process, the researchers acknowledged.
"However, the code was not optimized," they wrote. "It can be accelerated considerably with general-purpose graphics processing unit programming and by extracting only the discriminating features defined in this study."
The system also required an operator to manually draw ROIs, but recent advances in automated segmentation algorithms could eliminate that requirement, according to the researchers.