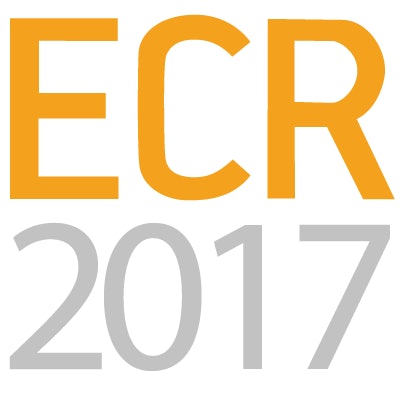
VIENNA - Deep artificial neural networks can read mammograms as well as radiologists, according to research presented at ECR 2017 on Thursday.
Whether radiologists welcome the finding as good news remains to be seen, as the field continues to grapple with the implications of artificial intelligence. But in any case, mammography is a good candidate for machine learning, said presenter Dr. Anton Becker of the University of Zurich.
"Mammography interpretation is a challenging task, and the performance of even experienced radiologists is only moderate," Becker said. "Mammographic images are an ideal target for computer-based analysis because they're 2D projection images and they're very high-resolution images, so there's a lot of information to feed to the computer."
Industrial grade
Becker and colleagues sought to evaluate the diagnostic performance of an industrial-grade deep-learning artificial neural network for the detection of breast cancer. The network is based on artificial neurons that are stacked in layers, much like natural neurons; the different layers extract different features from test images, according to Becker.
"One big advantage of the network we used is that it generates heat maps that highlight what the neural network sees as suspicious," he told session attendees.
For the research, the group included 3,271 mammograms taken in 2012 at the University of Zurich. Becker and colleagues identified 143 patients diagnosed with cancer; of these, 90 were invasive ductal carcinoma, 13 were ductal carcinoma in situ, 13 were invasive lobular carcinoma, and three were mucinous carcinoma.
"In the group that had lesions, we excluded patients who had prior procedures because it might train the neural network for hematomas or scars, which could produce a falsely good performance," he said.
The research was conducted in two studies. The first included the 143 cancer cases, matched with controls taken from the same dataset for breast density and patient age; the neural network was trained with these two cohorts. For this first study, Becker also selected patients with cancer and a matched control group from another, publicly available dataset, which he and colleagues used to test the performance of the trained neural network using receiver operating characteristic (ROC) analysis. The second study used the University of Zurich exams taken during the first nine months of the research time frame to train the neural network, and those taken the last three months to test it.
For both studies, three radiologists with three, seven, and 10 years of mammography experience, respectively, evaluated both datasets. Becker and colleagues then compared the area under the ROC curves (AUC) between these readers and the neural network.
In the first study, the AUC for the neural network was 0.81. One of the three radiologist readers showed comparable performance, with an AUC of 0.83, while the other two performed better than the neural network, with AUC values of 0.91 and 0.94. In the second study, the neural network's performance was comparable to the radiologists' performance, with an AUC value of 0.82, compared with the radiologists' range of 0.77 to 0.87.
"In the second study, we found that the readers showed higher specificity, but the neural network showed higher sensitivity," Becker said.
Quick learner
The findings suggest not only that neural networks are capable of performing on par with radiologists, but also that they can be trained with a relatively small amount of data, Becker said.
"Our research shows that deep neural networks can be trained with about 140 cases," he said. "Up to now, studies have used much larger datasets."
And despite being an all-purpose neural network, rather than one specifically designed for medicine, much less mammography, the computer learned quickly, Becker noted.
"This was a general-purpose network, and already it achieved comparable accuracy to experienced radiologists," he said.
Becker conceded that machine learning still has a ways to go before it can outperform humans -- and there are a variety of factors that still need to be incorporated into the technology.
"Integrating concepts like multiple views, laterality, and lesion evolution into next-generation neural networks may be useful," he concluded.