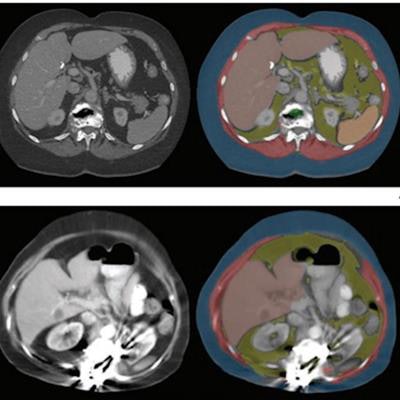
Artificial intelligence (AI) tools for assessing body composition on CT appear to produce highly accurate technical results, according to research published February 22 in the American Journal of Roentgenology.
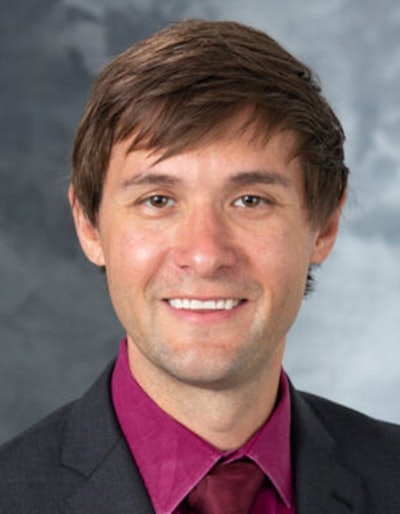
The findings could translate into better patient care, wrote a team led by Dr. B. Dustin Pooler of the University of Wisconsin in Madison.
"Given that over 70 million CT examinations are performed annually in the United States, these tools have tremendous potential to be used for opportunistic health screening on abdominal CT examinations obtained for nearly any indication," the team noted.
A concern about AI assessment tools is whether their performance is consistent across imaging studies conducted at various facilities and equipment types, Pooler and colleagues wrote. To address this concern, the investigators tested the technical performance of a set of three automated tools for AI analysis of abdominal body composition (bone attenuation, muscle composition and attenuation, and amounts of visceral and subcutaneous fat) on a set of CT exams taken at different institutions and on different scanners.
Their study included 8,949 patients who underwent 11,699 abdominal CT exams; the exams were conducted at 777 different institutions on 82 scanners from six different manufacturers. Pooler and colleagues evaluated one axial series of images per exam for the three AI tools' performance in assessing body composition. It defined "technical adequacy" as tool output values within established reference ranges and "failure" as values outside of the reference range.
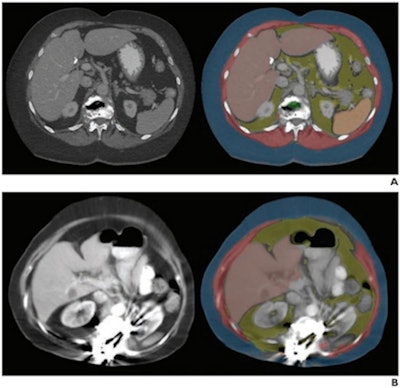
The group reported that the three automated AI tools for measuring body composition were technically adequate in 97.7% of the CT exams. The failure rate was 2.3%, and these failures were primarily (88%) due to an image processing error caused by incorrect DICOM header voxel dimension information (i.e., an anisometry error).
Performance of three types of AI tools for assessing body composition from CT images | |
Type of tool | Technical adequacy rate |
Bone attenuation | 97.8% |
Muscle amount and attenuation | 99.1% |
Visceral and subcutaneous fat amounts | 98% |
The authors acknowledged some limitations of the study, including the fact that they only evaluated technical adequacy and did not assess the ability of these AI tools to predict and influence clinical outcomes.
In the end, how can AI body composition assessment tool failures be mitigated? By hewing to AI protocols, the team advised.
"AI tool failure relating to technical factors may be largely preventable through proper acquisition and reconstruction protocols," the researchers concluded.