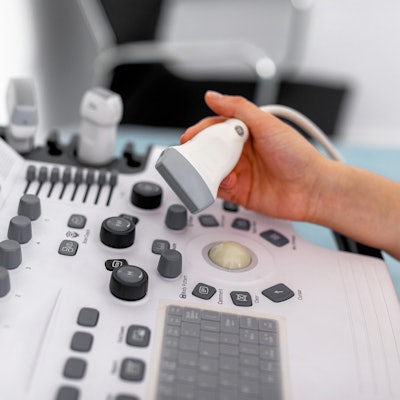
A deep-learning radiopathomics model using preoperative ultrasound images and biopsy slides can differentiate between luminal and nonluminal breast cancers at early stages, results published July 19 in eBioMedicine showed.
Researchers led by Yini Huang, MD, from the Collaborative Innovation Center for Cancer Medicine in Guangzhou, China, highlighted the success of their model, which outperformed a deep-learning radiomics model based on ultrasound images only and a deep-learning pathomics model based on whole-slide images.
"The superior performance of the [radiopathomics] model showed the potential for application in tailoring neoadjuvant treatment for patients with early breast cancer," Huang and colleagues wrote.
Neoadjuvant chemotherapy is recommended for treating nonluminal breast tumors. This makes distinguishing nonluminal from luminal tumors important for guiding treatment strategies. However, the researchers pointed out that molecular immunohistochemical subtypes based on biopsy tissue don't always match final results based on tissue taken from surgery.
Huang and colleagues wanted to develop and validate their model, which uses preoperative ultrasound images, as well as hematoxylin and eosin (H&E)-stained biopsy slides. They wrote that the model's purpose is to preoperatively differentiate between luminal and nonluminal breast cancers at early stages.
The researchers tested the model in a prospective, multicenter study. For the study, they collected 1,809 ultrasound images and 603 H&E-stained whole slide images from a total of 603 women with early-stage breast cancer.
The researchers constructed the radiopathomics model based on feature sets including deep- learning analysis of ultrasound, deep-learning analysis of whole-slide images, and an ultrasound-guided coattention module for fusing ultrasound and whole-slide images.
The team also compared the diagnostic efficacy of the model with that of a deep-learning radiomics model and deep-learning pathomics model in the external test cohort.
The group found that the radiopathomics model showed an AUC value of 0.929 in the internal validation cohort, which consisted of 342 ultrasound images and 114 whole-slide images from 114 patients. Additionally, it outperformed models only using ultrasound or whole-slide images alone in the external test cohort consisting of 279 ultrasound images and 90 whole-slide images from 90 patients.
Performance of models in distinguishing luminal from nonluminal breast cancers | |||
Ultrasound images-only model | Whole slide images-only model | Radiopathomics model | |
AUC | 0.815 | 0.802 | 0.9 |
Additionally, the model showed high positive predictive value in the test set at 0.957.
The researchers suggested that the high performance of their radiopathomics model could be due to its integration of heterogeneous radiomics and pathomics features. This combined approach captures micro- and macrostructural features to predict the hormonal receptor expression of breast cancer.
They added that they hope to incorporate more types of whole slide images to further train their model.
"The [radiopathomics] model can assist clinicians to classify luminal and non-luminal lesions rapidly. Input the routinely available images of breast ultrasound and biopsies to the... model would obtain a prediction result," the study authors wrote. "Therefore, decisions regarding whether neoadjuvant therapy should be given can be at the discretion of the model."
The study can be found in its entirety here.