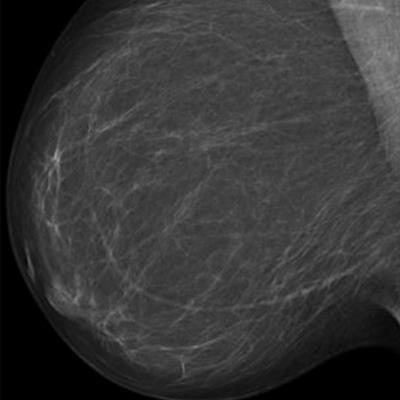
A combined diagnostic AI system and mammographic texture model can improve risk assessment for interval breast cancer and long-term breast cancer, a Danish study published August 29 in Radiology found.
Researchers led by Andreas Lauritzen, PhD, from the University of Copenhagen found that this combined method also helped identify women at high risk of breast cancer.
"The combination model in our study, using the examination score and texture risk, achieved a higher area under the curve [AUC]... than the Gail or Tyrer-Cuzick models, within a comparable follow-up period," Lauritzen and colleagues wrote.
Risk-stratified screening is used to better assess what resources are needed to screen and treat women with breast cancer. Some risk models that are used include the Gail and Tyrer-Cuzick models, which show that clinical risk factors can predict five-year, 10-year, and lifetime risk. BI-RADS meanwhile takes additional risk factors into account, such as breast density and genetic factors.
However, the researchers pointed out that these models require "extensive" data collection methods, which "complicate" using them in screening practices.
Lauritzen and co-authors investigated whether a commercially available diagnostic AI system (Transpara, version 1.7.0, ScreenPoint Medical) combined with a texture model consisting of mammography images could improve risk assessment. The AI model specializes in predicting short-term risk while the texture model predicts long-term risk. The team also aimed to evaluate the combination model's performance when age and percentage mammographic density were included.
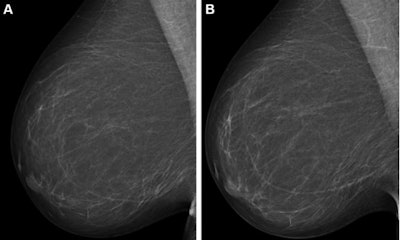
The researchers trained the combination model on mammography exam data taken from 39,345 Dutch women; the researchers also used retrospective data from 119,650 Danish women who underwent consecutive screening mammography between 2012 and 2015 and at least five years of follow-up data.
The team found that the combination model achieved a higher AUC for interval and long-term cancers grouped together than either system separately.
Performance of combination model compared to separate methods | ||||
Measure | AI system | Texture model | Combination model | p-value* |
AUC | 0.7 | 0.66 | 0.73 | < 0.001 |
The researchers reported that the 10% of women with the highest combined risk identified by the combination model consisted of 141 of 320 (44.1%) interval cancers and 472 of 1,401 (33.7%) long-term cancers in the study group.
The team also found that the combination model achieved a sensitivity of 36.5% for interval and long-term cancers together. They noted that this was higher than the sensitivities for texture risk alone (24.5%, p < 0.001) and the examination score alone (33.9%, p < 0.01).
Finally, the group found that the combination model that also included age and percentage mammographic density achieved a sensitivity of 36.1%, although this was not significantly different from the combination model that did not incorporate these characteristics (p = 0.66).
Future studies should further investigate whether the combination model adapts well to other mammographic devices and institutions, according to the researchers.
"Additional research should focus on translating combined risk to lifetime or absolute risk for comparison with traditional models," they wrote.
In an accompanying editorial, Clare Poynton, MD, PhD, and Priscilla J. Slanetz, MD, both from Boston University, wrote that while AI-based tools such as in the Lauritzen study have potential, it's critical to conduct more research on multiple risk algorithms in large diverse imaging data sets so that risk assessment is "appropriately directed toward populations who will benefit most from a more personalized approach to early breast cancer detection."
The full study can be found here.