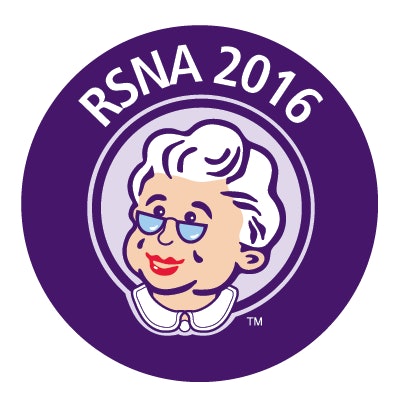
CHICAGO - A deep-learning method offers potential for providing a "human-like" diagnosis on chest x-rays, a group from the U.S. National Institutes of Health (NIH) Clinical Center reported this week at the 2016 RSNA meeting.
Following up on previous work to detect a number of common diseases from patient scans, the NIH team sought to explore the possibility of automatically generating a more comprehensive and human-like diagnosis. After being trained on a database of chest x-rays and report summaries that had been annotated using standardized terms, the resulting deep-learning framework offers a promising first step toward automated understanding of medical images, according to the group.
"The trained system can not only detect a disease from a chest x-ray, but it can also describe its context, such as its size, location, affected organ, severity, etc.," said senior author Dr. Ronald Summers, PhD.
The researchers shared their findings in a poster presentation at McCormick Place.
Previous limitations
Research and applications for computer-aided detection (CAD) have so far been limited to detecting diseases for which they have been specifically targeted and trained, according to Summers.
"Nonetheless, there exists a vast amount of electronic health records and images in PACS from which a more 'general' framework for training an advanced CAD [software] can be developed," he said.
To train, validate, and test deep-learning systems comprised of convolutional neural networks (CNNs) and recurrent neural networks (RNNs), the group used a publicly available database of 7,283 chest x-rays and 3,955 report summaries. These were annotated using Medical Subject Headings (MeSH) standardized terms based on predefined grammar. Summarizing the reports with the standardized terms helps remove the ambiguity found in radiology reports, according to the group.
The CNNs were trained with image disease labels that were mined from the annotations, while the RNNs were trained to generate the entire MeSH annotations with the disease contexts, according to the team. The researchers, who also included presenter Hoo-Chang Shin, PhD; Kirk Roberts; and Le Lu, PhD, noted that automatic labeling of images can be improved by jointly taking into account image and text contexts.
Testing performance
The project utilized 217 MeSH terms to describe a disease, with a range of 1 to 8 terms (mean, 2.56; standard deviation, 1.36) for each image. The researchers used 6,316 images to train the system. Validation was performed using 545 images, and testing was conducted on 422 images.
Rates for predicting words matching true annotations | |||
No. of consecutive words | Training | Validation | Testing |
1 | 90% | 62% | 79% |
2 | 62% | 30% | 14% |
3 | 79% | 14% | 5% |
The results show that a general framework for generating image captions can be adopted to provide a more "human-like" diagnosis on chest x-rays, according to the group.
"While we demonstrate how we can get closer to a more 'human-like' and 'general' diagnosis system, more research needs to be done to improve its performance," Summers noted.