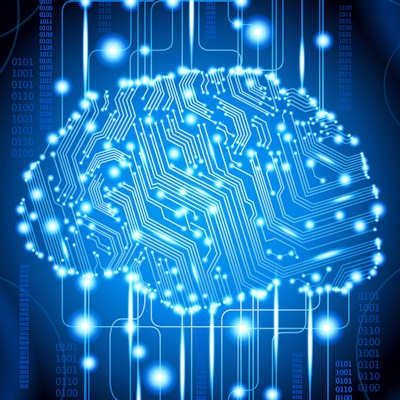
While artificial intelligence (AI) is being touted as the technology of the future, there are a number of barriers that are likely to hinder its broader adoption in radiology, ranging from tools for training algorithms to regulatory concerns at the U.S. Food and Drug Administration (FDA).
One of the biggest stumbling blocks is the lack of large, well-annotated datasets to train AI algorithms, according to Dr. Raymond Geis of the University of Colorado School of Medicine.
Indeed, there are no reliable sources of curated data that replicate clinical environments, agreed Dr. Luciano Prevedello from Ohio State University Wexner Medical Center.
"Most of the research performed today in this area either relied on painful manual review and curation of the images in the research setting or relied on previously acquired and curated data for a specific clinical trial -- typically focused on a specific topic with well-defined inclusion and exclusion criteria and not necessarily representative of the entire patient population," Prevedello said. "Institutions will need to work more collaboratively to overcome this issue."
Nearly every institution is sitting on a huge amount of data in its image archives, but it's all very much raw material that needs to be extracted, cleaned, and refined before it can be used to train deep-learning algorithms, said Dr. Marc Kohli of the University of California, San Francisco (UCSF).
"Institutions and companies that build effective pipelines of data will succeed," Kohli said. "The next biggest challenge will be attempting to generalize these pipelines and practices across several institutions. Without things like shared procedure codes in wide use -- such as the Logical Observation Identifiers Names and Codes (LOINC) and the RSNA's RadLex Playbook -- even basic semantic translation [of data in between institutions] is limited."
The regulatory process also represents a considerable hurdle to the adoption of new AI algorithms in radiology, according to Dr. Eliot Siegel of the University of Maryland.
"The 'black box' nature and the rapid growth of machine-learning applications will make it difficult for the FDA to keep up with submissions with regard to volume and the complex nature of testing and verification of machine-learning applications," Siegel said. "Additionally, the FDA does not currently have a good mechanism to allow PACS/workstation vendors to provide machine-learning algorithms written by developers outside of their own company due to the rigorous requirements for the process of software coding and documentation and testing."
Another issue is the computing requirements necessary for machine learning, which is best provided and scaled using cloud computing resources, according to Siegel. However, most radiology departments have been slow to use and trust the cloud, and there will also be pushback by IT departments unfamiliar with the technologies and the new companies offering these services, he said.
Dr. Bradley Erickson, PhD, of the Mayo Clinic in Rochester, MN, believes that the biggest barrier to rapid AI adoption is the number of scientists who believe that only hypothesis-driven research is science. Research grant applications have been rejected because researchers aimed to use deep-learning technology to discover markers for disease such as in radiogenomics, he said.
"The reviewers felt that it was science only if there was a hypothesis," Erickson said. "This type of thinking forces us into the evolutionary way of doing things and means radiology will not be as effectively engaged in revolutionary changes such as are envisioned by the Biden Cancer Moonshot initiative. In short, radiology needs to embrace discovery science."
Of course, radiologists must also be able to easily access these algorithms during their daily work.
"Despite the over 10,000 machine-learning algorithms that have been presented or described in the literature for diagnostic imaging, virtually none are available to radiologists at their workstation," Siegel said. "It will be critical to find a means of delivering these algorithms to the radiologist or clinician. Since they have been written using many types of software and on many types of systems and are often not optimized for performance, it will be a challenge to create unifying platforms for delivery of this software and to make it easy for those writing [machine-learning] algorithms to get their software to the marketplace."
Effect on radiology
Kohli doesn't believe that AI will replace radiologists. But he does believe it will change their jobs dramatically.
"I doubt that in 10 to 20 years radiology will look very similar to the radiology of today," he said. "In all honesty, this will be similar to the transformation that radiology has seen with PACS and advanced modalities like MR and PET."
While machine learning won't take over from radiologists anytime soon, it will provide more information from imaging exams, according to Geis.
"At some point, perhaps over 10 years from now, [machine learning] will do much of the image interpretation work radiologists do now," Geis said. "Radiologists then will need new computer software management skills and will spend more time running an ecosystem of hundreds of [machine-learning] algorithms."
AI will have a major effect on the practice of radiology -- increasingly so over time, Siegel said.
"We radiologists will expect far more in the future from our image display and information and dictation and tracking systems with regard to automation and 'intelligence,' " Siegel said. "Recommendations will be automatically tracked and clinical data will be presented with accompanying probabilities for disease using genomic data, clinical data, imaging data, lab data, etc. Relatively repetitive tasks such as the search for a rib fracture or lung nodules or pulmonary emboli on CT, [maximum standardized uptake value (SUVmax)] assessment on PET, and multisequence MRI analysis and comparison will be automated."
Siegel said AI software will act, in many ways, as a spelling or grammar checker of sorts. Instead of making the diagnosis, identifying all of the findings, or making recommendations, AI algorithms will make the interpretation process more efficient and less stressful for the radiologist, he said.
Even though people don't realize it, AI is already here, Prevedello said. The problem is that it's still limited to a few applications and clinical scenarios.
"What will happen is that ... our systems will become gradually smarter," Prevedello said. "Additional clinical applications will start popping up here and there. I believe that in the future, we will have a hard time determining the turning point when AI really became part of our work. We will just say, 'How were we able to work without it?' "
The immediate impact of AI on radiology will be to identify disease markers that are not perceived today, Erickson said. The second greatest effect will be a significant increase in quantitative reporting, as AI software can help overcome the burden of producing quantitative metrics on a routine basis, he said.
"Soon thereafter, they will produce structured preliminary reports utilizing this quantitative data," Erickson said. "This will significantly increase the use of structured reports, and likely lead to more effort to standardize the type of information that should be reported for the various types of examinations."
Should radiologists fear or welcome AI?
AI is an extremely exciting technology that radiologists should welcome, Prevedello said.
"It will not only allow us to be more comprehensive, precise, and quantitative in our imaging analysis, but it will also free us up more to spend more time with our patients," he said.
Education is the main way for radiologists to get prepared for AI, Prevedello said.
"AI is not bulletproof," he said. "As with any other tool, you need to understand what it is doing to know its limitations."
Radiologists will need to learn to manage, implement, and evaluate machine-learning products and algorithms, Geis said.
Kohli noted that it's a natural human tendency to fear the change that any technology brings.
"Ultimately, the decision to fear change or embrace it comes down to the ability of the individual to see past the hype and find comfort with redefining their future," Kohli said.
Radiologists should both fear and welcome AI, according to Erickson.
"If those who feel AI is not science have their way, then nonscientific entities will develop radiology and implement it," he said. "This is happening today. That will not be pretty for radiology, medicine, or patients."
On the positive side, deep learning is already showing that it can make diagnoses that are not possible today, Erickson said.
"Deep learning could be a gold mine of information that puts radiology in an even more important role for patient care," he said. "But that will only happen if we are leading the effort. If we try to suppress or ignore AI, those who think they understand radiology will replace us with their perception of radiology."
AI should be welcomed and embraced by radiologists, Siegel said.
"Imaging studies will continue to grow in volume and complexity, and there will be more pressure to be accurate, safe, and efficient, as well as to communicate findings clearly and effectively," he said. "AI technology in radiology will become ubiquitous and errors such as laterality (body of report says left but impression says right, for example), missed fracture, missed lung nodule, lack of communication of a critical finding, egregious transcription error, and many others will become as anachronistic as 'glass plates' and film in our specialty."