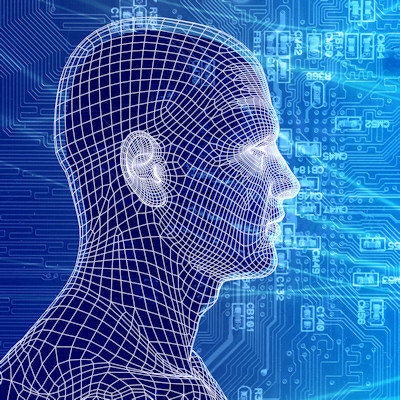
Artificial intelligence (AI) in medical imaging is on the rise and already becoming part of the standard of care. But with all the hype, one must ask: Is next-generation imaging AI simply a new take on computer-aided detection/diagnosis (CAD)? Are we witnessing an evolution, or perhaps a revolution?
This article gives five reasons why next-generation imaging AI is fundamentally different from traditional CAD, and it will show how AI is an entirely new paradigm in computer-aided imaging. There are two components to this new paradigm: deep learning as the core technology, and an evolving workflow integration.
These two components are far from becoming a commodity, but AI solutions based on them will revolutionize the types of products made available to radiologists.
1. Next-gen AI solutions can be like a personal assistant.
Let's begin with a basic truism: The new generation of AI solutions is proving to be more accurate than traditional CAD (in some cases, by a huge margin). Several breakthroughs in AI technology, mainly the deep-learning revolution, the increase in medical AI research, and the opening of large annotated datasets, have led to significant improvements in the levels of accuracy in AI algorithms.
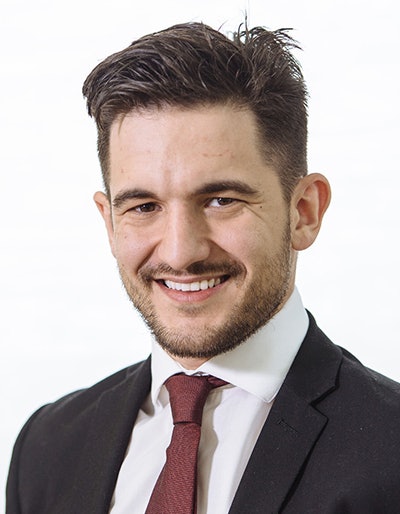
Moreover, deep-learning technologies are designed to interpret large amounts of data, learn from mistakes, and improve over time. This makes them not only more accurate but also actually more robust to changing circumstances, including different types of scanners and diverse patient populations (provided that the training data are available).
There are even systems that have reached a performance level that almost matches that of a radiologist (albeit on very narrow use cases). We are reaching the point where new-generation AI systems are sensitive enough to detect minute image changes that are challenging to most human eyes.
In fact, the biggest leap of AI is actually in the improved specificity (reduced false-positive rate) of these solutions. This, in turn, allows a much smoother user experience. The result is that analysis speed is increased, time to treatment is accelerated, and diagnostic quality is improved.
Great strides in AI accuracy have been demonstrated recently by an ophthalmologist at the University of Iowa, who developed software that uses AI to detect diabetic retinopathy without a person interpreting the results. Now cleared by the U.S. Food and Drug Administration, IDx-DR exceeded all prespecified superiority end points in sensitivity, specificity, and imageability -- proving that, in theory, the accuracy of such systems could be on par with that of a trained radiologist.
2. Next-gen AI solutions have comprehensive capabilities.
There is a second dramatic benefit to companies that adopt deep learning as their core technology. Deep-learning systems are not only more accurate but also much more scalable. If a company builds the right infrastructure, it can utilize the same engine that detects intracranial hemorrhages to detect cervical spine fractures or pulmonary embolisms, resulting in broad coverage of radiology workflow.
In contrast, typical CAD systems tend to be limited to detecting or diagnosing a specific disease (e.g., lung or breast cancer). With such a narrow scope, designed to deliver just a single answer, and due to the underlying technology limitations, it is difficult for CAD systems to expand the way in which deep-learning solutions have.
Current AI developers are using machine-learning programs, powered by complex deep-learning pipelines with scalable, immersive diagnostic engines, to enable the detection of a broad range of pathologies. By feeding AI algorithms large volumes of data and teaching them to interpret and learn new concepts and rules, AI can evolve over time and improve medical diagnosis.
3. Next-gen AI solutions offer seamless workflow integration and "always-on" AI.
Traditional on-demand AI systems that are available today require physicians to actively request the intervention of the AI algorithm. Due to increased standardization, next-gen AI systems can have a much tighter workflow integration.
For instance, hospitals can now enjoy "always-on" AI, running continuously in the background and assisting in interpreting scans. These AI systems eliminate the need to click on anything to request a task. Instead, they sift quickly and automatically through each patient scan, pinpointing urgent findings and sparing radiologists from drowning in vast amounts of irrelevant data.
Next-gen AI imaging not only integrates easily into the radiologist's work environment -- including PACS software used predominantly by radiologists -- but also actually improves efficiency without altering the existing workflow.
Historically, some CAD systems have been criticized as being inefficient due to their design as standalone systems. Each CAD application included complex functionality methods and had to be installed on a dedicated workstation and, therefore, could not be integrated into the radiologist's workflow.
Even if they were not in a different workstation, most CAD systems required a dedicated workflow, encroaching on the already limited real estate that exists on a radiologist's workstation. One hospital actually showed me its "CAD basement," where many old computers intended for different CAD applications had been collecting dust for years.
The American College of Radiology Data Science Institute (ACR DSI) is pushing to set new industry standards for the practice of radiology. Due to the opening up of proprietary software and vendor communication (supported by the ACR DSI), it is now possible -- for the first time -- to deploy AI within the existing workflow environment.
4. Next-gen AI solutions offer sophisticated output.
In the past, the traditional CAD approach treated medical images as pictures intended solely for visual interpretation, using arrows and other markings applied to the image.
Since then, advanced systems are utilizing imaging-based AI to deliver complex output with new capabilities. For example, after reviewing a scanned image, IBM Watson's electronic health record-driven patient synopsis prioritization solution can pull medical history and present a comprehensive view of the patient's condition.
In the foreseeable future, machine-learning software could provide a preliminary diagnosis of diseases and determine the optimal next step in narrowing differential diagnosis. In the future, these new systems could, potentially, combine imaging with clinical and genomic data. Increased capabilities of such radiomics-based systems may enable drug efficacy prediction, allowing treatment optimization for each individual patient.
In parallel, AI systems may analyze medical literature to identify the most relevant references for the given case. The way is open to the truly intelligent clinical assistant.
5. Next-gen AI solutions provide value across the entire clinical workflow.
New integration standards are appearing and, with them, new ways AI can improve the radiologist's day-to-day workflow. In the past, traditional CAD systems provided only limited diagnostic support. For next-generation AI, this is no longer true.
For example, in prioritization, some next-gen AI solutions (CADt) have shown the ability to assist in prioritizing time-sensitive cases. Other systems can provide quantification of white-matter lesions.
AI can now help with scheduling, patient management, report generation, and workflow optimization. It can also help choose the right imaging approach for the specific clinical context and even reduce operator dependency.
The broader the workflow integration, the greater the potential. With new standards emerging, we're only now beginning to scratch the surface.
The new paradigm
As the field of radiology continues to evolve from traditional CAD systems to next-gen AI, it will soon be hard to imagine practicing radiology without the assistance of these new AI partners, which will take on a significant portion of the workload. They will, most likely, become a standard of care for diagnostic examinations in daily clinical work, which will help radiologists focus on diagnosis and communication with patients and other physicians.
What started as an incremental technological improvement morphed into a qualitative breakthrough. Now we have at our fingertips the ability to combine a wide variety of data sources, including diagnostic imaging, clinical information, and genetic data. Based on AI analysis of this diverse information, we can attain new services such as quantitative follow-up, risk assessment, and decision support in diagnostic and patient management processes.
AI systems can even help evaluate the efficacy of various drugs and other treatments. Imagine all these services seamlessly integrated into the radiologist's workflow. True, many of these advanced features are still on the drawing board rather than in common clinical practice, but with recent technological breakthroughs, the door has been opened and the ball is rolling.
Elad Walach is the co-founder and CEO of Aidoc, a healthcare AI start-up focused on using deep learning to relieve the bottleneck in medical image diagnosis. Walach began his career in the elite Israeli Defense Forces' Talpiot technology program. He served as a researcher in the Israeli Air Force's algorithmic division, where he raised through the ranks, reaching the position of algorithmic research leader. He led several teams focused on machine-learning and computer-vision projects from inception to execution. Walach holds a Bachelor of Science in mathematics and physics from the Hebrew University of Jerusalem and a Master of Science in computer science with a focus on deep learning from Tel Aviv University.
The comments and observations expressed are those of the author and do not necessarily reflect the opinions of AuntMinnie.com.