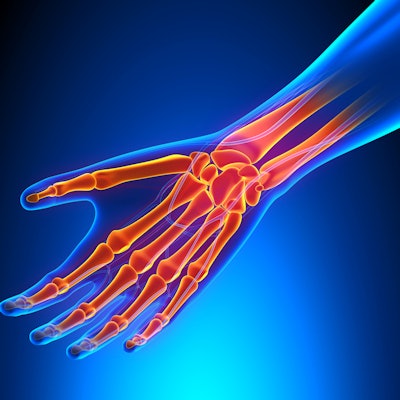
An artificial intelligence (AI) algorithm can both detect and show the position of fractures on wrist radiographs with a high level of sensitivity and specificity, according to research published online January 30 in the inaugural issue of the RSNA's new Radiology: Artificial Intelligence journal.
Researchers from the National University of Singapore trained a convolutional neural network (CNN) to identify as well as localize radius and ulna fractures on the frontal and lateral views of wrist radiographs. In testing, the model yielded more than 98% sensitivity and 73% specificity on a per-study basis, according to Dr. Yee Liang Thian and colleagues.
"The ability to predict location information of abnormality with deep neural networks is an important step toward developing clinically useful artificial intelligence tools to augment radiologist reporting," the researchers wrote.
Missed fractures on radiographs in the emergency department represent one of the most common causes of diagnostic errors and litigation. A few research studies have demonstrated the feasibility of CNNs for this application, but these efforts simply classified radiographs into either fracture or nonfracture categories and did not localize the actual region of the abnormality, according to the researchers.
"It is difficult for clinicians to trust broad classification labels of such 'black-box' models, as it is not transparent how the network arrived at its conclusion," the group wrote. "Location information of the abnormality is important to support the classification result by providing visual evidence that is verifiable by the clinician."
As a result, the researchers sought to explore the feasibility of an object-detection CNN, a type of deep-learning model that can detect objects and draw bounding boxes around them on images. The researchers first extracted 7,356 wrist radiographic studies -- 7,295 frontal images and 7,319 lateral images -- from their hospital's PACS.
One of three radiologists then reviewed and annotated each of the images -- including drawing bounding boxes of fractures. These studies were then used to train a deep-learning model based on the Inception-ResNet version 2 Faster R-CNN architecture. Of the 7,356 studies, 90% were used for training the CNN and 10% were used for validation.
Next, the researchers tested the model on 524 consecutive emergency department wrist radiographic studies and compared its findings with a reference standard of two radiologists reading in consensus. The model detected and correctly localized 310 (91.2%) of the 340 fractures seen on the frontal views and 236 (96.3%) of the 245 fractures found on the lateral views.
CNN performance for analyzing wrist fractures on x-rays | |||
Frontal view | Lateral view | Per study | |
Sensitivity | 95.7% | 96.7% | 98.1% |
Specificity | 82.5% | 86.4% | 72.9% |
Area under the curve (AUC) | 0.918 | 0.933 | 0.895 |
Delving further into the data, the researchers found that the algorithm didn't produce significant differences in AUC between pediatric and adult images, or between images from patients with and without casts. However, the neural networks were significantly more sensitive for displaced fractures compared with minimally or undisplaced fractures on both frontal (p = 0.005) and lateral (p = 0.01) views.