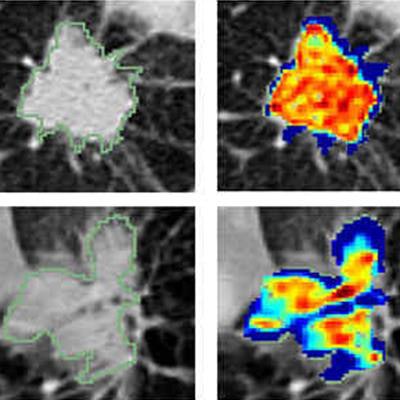
Artificial intelligence (AI) and radiomics can identify which non-small cell lung cancer (NSCLC) patients are likely to respond to chemotherapy, potentially enabling likely nonresponders to be shifted to alternative treatments, according to research published online March 20 in Radiology: Artificial Intelligence.
A multi-institutional research team found that a machine-learning classifier trained using radiomic texture features that were extracted from within and around the nodule on baseline CT scans could predict response to chemotherapy. What's more, these features were also significantly associated with time to progression as well as overall survival.
"Our results showed clear evidence of the role of peritumoral texture patterns in predicting response and time to progression after chemotherapy," said co-first author Mohammadhadi Khorrami, a PhD student at Case Western Reserve University School of Engineering, in a statement from the RSNA.
Although platinum-based chemotherapy is typically the first-line treatment of advanced-stage NSCLC, only approximately one in four patients responds well to the treatment, and unfortunately, it's not currently possible to ascertain which patients will benefit, according to the researchers. As a result, they sought to determine if radiomics texture features both within and without the nodule could be used to accurately predict chemotherapy response, time to progression, and overall survival in these patients.
The researchers retrospectively analyzed 125 patients with NSCLC who had received pemetrexed-based platinum doublet chemotherapy at the Cleveland Clinic. Of the 125 patients, 53 were placed in a training set of patients, and 72 were included in a validation set. Each set had approximately an equal number of responders and nonresponders to treatment. Next, the researchers trained a machine-learning classifier to predict response to chemotherapy by using radiomic texture features extracted from the intra- and peritumoral regions of noncontrast-enhanced CT images.
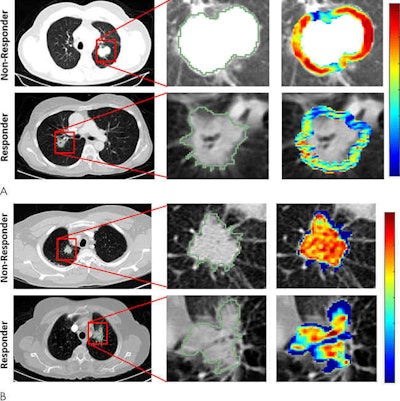
The combination of radiomics features with a quadratic discriminant analysis classifier produced a mean area under the receiver operating characteristic curve (AUC) of 0.82 in the training set and 0.77 in the independent testing set for predicting response to chemotherapy. Furthermore, the radiomics risk-score signature -- calculated via regression analysis -- was significantly associated with time to progression (hazard ratio, 2.8; p < 0.0001) and overall survival (hazard ratio, 2.35; p < 0.0011).
"Additionally, decision curve analysis demonstrated that in terms of clinical usefulness, the radiomics signature had a higher overall net benefit in prediction of high-risk patients to receive treatment than the clinicopathologic measurements," the authors wrote.
The study is the first to demonstrate that computer-extracted patterns of heterogeneity from outside of the tumor were predictive of chemotherapy response, according to co-first author Dr. Monica Khunger of the Cleveland Clinic.
"This is very critical because it could allow for predicting in advance of therapy which patients with lung cancer are likely to respond or not," she said in a statement. "This, in turn, could help identify patients who are likely to not respond to chemotherapy for alternative therapies such as radiation or immunotherapy."
The researchers noted that additional, large-scale multisite validation would be needed before the radiomic model could be deployed clinically.