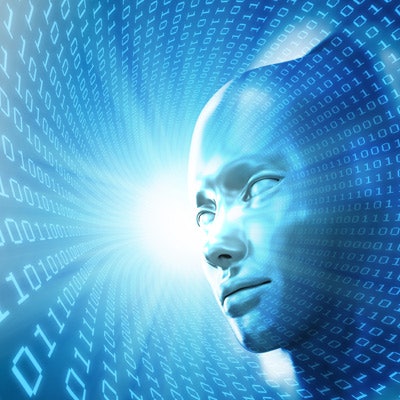
Two large public image datasets commonly used to train artificial intelligence (AI) algorithms have quality control (QC) issues that could potentially limit their utility, according to research published online November 6 in Academic Radiology.
Dr. Luke Oakden-Rayner of the Australian Institute for Machine Learning in Adelaide analyzed the quality of image labels for a subset of the ChestXray14 (CXR14) and Musculoskeletal Radiology (MURA) datasets. He found that the CXR14 image labels did not always reflect the visual content of the images. The MURA labels were more accurate, but some inaccurate labels were still found in cases with degenerative joint disease, according to Oakden-Rayner.
"The [positive predictive value] of the labels in the CXR14 dataset were typically quite low, even allowing for differences in reporting style and interobserver variability," he wrote. "By contrast, the MURA labels were of much higher accuracy, other than in the subset of patients with features of degenerative joint disease. In both datasets, the errors in the labels appear directly related to the weaknesses of the respective labeling methods."
To address the lack of large, well-characterized image datasets for training AI algorithms, several large datasets have been released in recent years. These include CXR14, a dataset of over 112,000 chest radiographs produced by a team of researchers at the U.S. National Institutes of Health Clinical Center, and MURA, a dataset from the Stanford Machine Learning Group that includes 40,561 upper-limb radiographs.
Oakden-Rayner, a board-certified radiologist, visually explored a subset of approximately 700 images from both datasets to assess the quality of the original labels. The CXR14 labels had positive predictive values that were mostly 10% to 30% lower than the values presented in the original documentation, according to the Oakden-Rayner.
"There were other significant problems, with examples of hidden stratification and label disambiguation failure," he wrote.
Oakden-Rayner found the MURA labels to be more accurate. However, the original normal or abnormal labels were inaccurate for the subset of cases with degenerative joint disease -- yielding only 60% sensitivity and 82% specificity.
The disconnect between the development of these public datasets and the usage of the data can lead to a variety of major problems, he noted.
"The accuracy, meaning, and clinical relevance of the labels can be significantly impacted, particularly if the dataset development is not explained in detail and the labels produced are not thoroughly checked," he wrote.
These problems could be mitigated by expert visual review of the label classes and documentation of the development process, strengths, and weaknesses of the dataset, according to Oakden-Rayner.
"This documentation should include an analysis of the visual accuracy of the labels, as well as the identification of any clinically relevant subsets within each class," he concluded. "Ideally, this analysis and documentation will be part of the original release of the data, completed by the team producing the data to prevent duplication of these efforts, and a separate test set with visually accurate labels will be released alongside any large-scale public dataset."