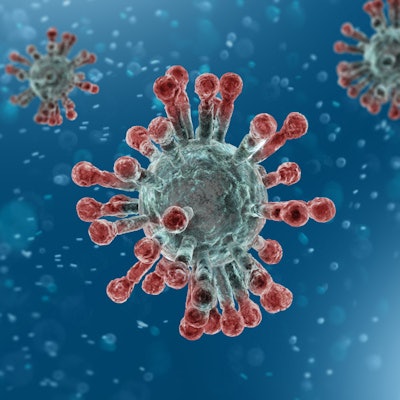
Canadian researchers are developing an open-source artificial intelligence (AI) algorithm called COVID-Net for detecting COVID-19 on chest x-rays. They've also made the training dataset available for other researchers.
Linda Wang and Alexander Wong of the University of Waterloo and AI software developer DarwinAI trained the COVID-Net algorithm using COVIDx, a dataset they assembled from two other open-source datasets containing nearly 6,000 chest radiographs. Their deep convolutional neural network yielded 100% sensitivity, 80% positive predictive value, and 83% accuracy for identifying COVID-19 on a small test set.
"By no means a production-ready solution, the hope is that the promising results achieved by COVID-Net on the COVIDx test dataset, along with the fact that it is available in open-source format alongside the description on constructing the open-source dataset, will lead it to be leveraged and [built] upon by both researchers and citizen data scientists alike to accelerate the development of highly accurate yet practical deep-learning solutions for detecting COVID-19 cases from chest radiography images and accelerate treatment of those who need it the most," the authors wrote in an article published online March 22 on arXiv.org.
The researchers built the COVIDx dataset by combining two publicly available datasets: a COVID-19 chest x-ray dataset and the Kaggle chest x-ray dataset for the pneumonia challenge. The resulting dataset included 5,941 posteroanterior chest radiography images from 2,839 patients. Due to the scarcity of available case data, there were only 68 radiographs from 45 COVID-19 patients in the dataset.
After COVID-Net was trained, it was then tested on a subset of the COVIDx dataset.
Performance of COVID-Net on test set | ||||
Normal cases | Bacterial infection | Non-COVID-19 viral infection | COVID-19 viral infection | |
Sensitivity | 73.9% | 93.1% | 81.9% | 100% |
Positive predictive value | 95.1% | 87.1% | 67% | 80% |
Despite the promising level of performance, the researchers acknowledged a number of limitations for COVID-Net. Although it provided 100% sensitivity for the eight COVID-19 cases in the test dataset, the number of positive COVID-19 cases were small in the dataset compared with other infection types. The algorithm also had two false positives -- misidentifying two patients who had bacterial infections.
What's more, COVID-Net offered a lower positive predictive value for non-COVID-19 viral infections cases and suffered from a noticeably lower sensitivity for normal cases.
"Therefore, based on these results, it can be seen that while COVID-Net performs well as a whole in detecting COVID-19 cases from chest radiography images, there are several areas of improvement that can benefit from collecting additional data as well as improving the underlying training methodology to generalize better across such scenarios," the authors wrote.
The researchers have made the source code and documentation for COVID-Net available on GitHub along with the dataset and the scientific paper.
"We are open sourcing this model to the community in hopes of developing a robust tool to assist health care professionals in combating the pandemic," said DarwinAI CEO Sheldon Fernandez in a post on Medium.