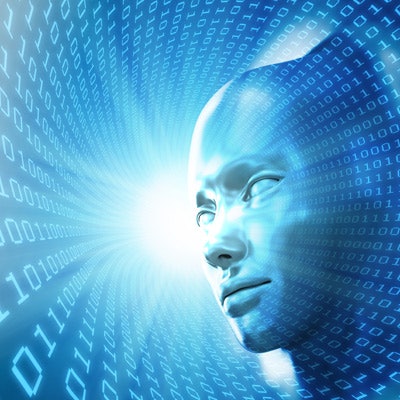
Artificial intelligence (AI) algorithms can differentiate normal and abnormal chest radiographs with an accuracy on par with experienced radiologists, enabling triage of these studies for priority review, according to research published online May 14 in npj Digital Medicine.
A team of researchers led by first author Yu-Xing Tang, PhD, and senior author Dr. Ronald Summers, PhD, of the U.S. National Institutes of Health (NIH) Clinical Center, found that several types of deep convolutional neural networks (CNNs) could achieve an area under the curve (AUC) of over 0.98 on an internal test set. They also found that a model could produce an AUC of 0.944 on an external test set.
"The remarkable performance in diagnostic accuracy observed in this study shows that deep CNNs can accurately and effectively differentiate normal and abnormal chest radiographs, thereby providing potential benefits to radiology workflow and patient care," the authors wrote.
After being pretrained on the ImageNet database and then trained on a dataset of approximately 8,500 radiographs from the NIH's ChestX-ray 14 dataset, the different deep CNN architectures -- AlexNet, VGG, GoogLeNet (Inception-v3), ResNet, and DenseNet -- were assessed on a hold-out test dataset of 1,344 radiographs from that NIH database.
Performance of AI for discriminating between normal and abnormal chest radiographs | ||||||
AlexNet | VGG-19 | Inception-v3 | ResNet18 | ResNet50 | DenseNet121 | |
AUC | 0.974 | 0.984 | 0.987 | 0.982 | 0.984 | 0.987 |
AlexNet achieved inferior results compared with the other models (p < 0.05), but the researchers found no significant differences amongst VGG-19, Inception-v3, ResNet18, ResNet50, and DenseNet121. As an example, ResNet18's performance included 94.6% accuracy, 96.5% sensitivity, and 92.9% specificity.
A reader study involving three U.S. radiologists with an average of nearly 30 years of experience was also performed on this same test dataset. The average interrater agreement between the consensus of the radiologists and the attending radiologist who had produced the original report was 98.4%, while the interrater agreement between the three readers was 94.8%.
Next, the researchers used one of the five equivalent models in experiments on a variety of external datasets. In testing on the RSNA's pneumonia detection challenge dataset, the VGG-19 model produced an AUC of 0.98, with an accuracy of 94.7%, 92.2% sensitivity, 96.3% specificity, 94.3% positive predictive value, and 95% negative predictive value for classifying chest x-rays as either being normal or showing pneumonia-like lung opacity.
Also, a VGG-19 model trained on the ChestX-ray 14 dataset produced an AUC of 0.944 on a test dataset of 432 chest radiographs from Indiana University Hospital, indicating the algorithm is likely to be highly generalizable, according to the researchers. Next, they found that an Inception-v3x model pretrained on cohorts of adult patients and then fine-tuned on pediatric patients yielded an AUC of 0.985 for classification of normal and pneumonia cases in a test set from Guangzhou Women and Children's Medical Center in China.
"This study shows that deep-learning models offer the potential for radiologists to use them as a fast binary triage tool, thus improving radiology workflow and patient care," the authors wrote. "In addition, this study may allow for future deep-learning studies of other thoracic diseases in which only smaller datasets are currently available. Taken together, we expect this study will contribute to the advancement and understanding of radiology and may ultimately enhance clinical decision-making."