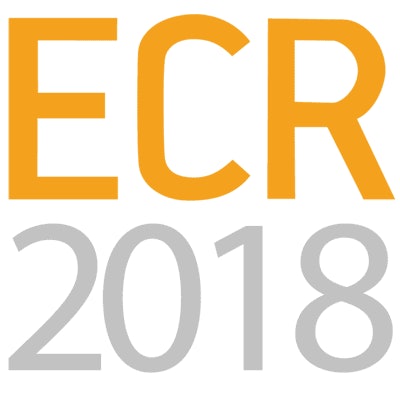
VIENNA - A deep-learning algorithm can accurately detect and measure the volume of intracranial calcifications, potentially facilitating research that could lead to new treatments for intracranial atherosclerosis, according to findings presented on Thursday at ECR 2018.
Researchers from Erasmus University Medical Center in Rotterdam, the Netherlands, have developed a deep-learning algorithm that yielded more than 85% accuracy in detecting intracranial calcifications. Calcification volume measurements produced by the automatic method also correlated highly with manual annotations by expert observers.
"This leads us to believe that our method can be used to speed up manual annotation of intracranial calcifications in large epidemiological studies and in clinical practice," said presenter Gerda Bortsova.
Marker for intracranial atherosclerosis
Intracranial calcifications are calcium deposits in the intracranial part of the carotid artery and are best visible in noncontrast CT. These calcifications are a marker for intracranial atherosclerosis, which is a major cause of stroke and related to dementia and cognitive decline, Bortsova said.
There is currently high demand for more research into the causes and consequences of intracranial calcifications. These studies could lead to the development of new treatments and preventive measures, she said.
However, studies investigating intracranial calcifications currently require manual labeling. In one approach to manual labeling, expert annotators delineate calcifications by manually choosing regions of interest around lesions, which are then identified by thresholding at more than 130 HU. In another approach involving visual scoring, readers subjectively estimate the severity of calcifications either in every slice or in the whole scan, Bortsova said.
"Both kinds of manual approaches are highly time-consuming, tedious, and also error-prone," she said. "That was our motivation as well [for developing] a fully automatic method for segmentation of intracranial calcifications."
Deep-learning method
The researchers trained a fully convolutional neural network using techniques such as deep supervision and dropout. They used 800 annotated image volumes from the Rotterdam Study for training the network, with 1,000 other annotated volumes set aside for validation.
In testing, the deep-learning method detected 86% of intracranial calcification voxels that were found in the Rotterdam Study; 17% of the detected voxels were not found by the expert observers.
"So that either meant that they were not calcifications or that the lesions were missed [by the observers], which happens quite often," Bortsova said.
The deep-learning method also performed well in measuring calcification volume -- an important factor that has been found to relate strongly to stroke risk. The correlation between automatic and manual volume measurements for intracranial calcifications yielded an intraclass coefficient correlation (ICC) of 0.98, which was comparable to an ICC of 0.99 for the human observers in the study.