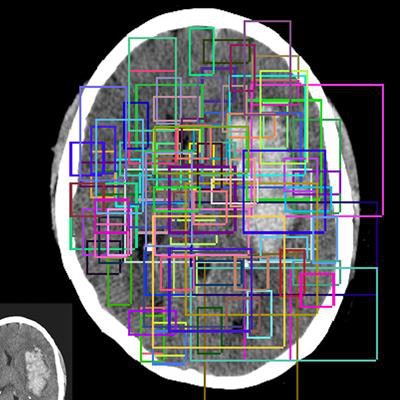
A deep-learning algorithm can provide high sensitivity and specificity for detecting brain hemorrhage on noncontrast head CT studies, according to research presented last week at the Society for Imaging Informatics in Medicine (SIIM) annual meeting in National Harbor, MD.
Dr. Peter Chang.
"Every other published algorithm is either optimized for high sensitivity (but with overdiagnosis) or high specificity (but with underdiagnosis)," he told AuntMinnie.com.
What's more, the algorithm has continued to provide high levels of accuracy since being placed into clinical use for noncontrast CT exams performed in UCSF's emergency room, Chang said.
The paper was one of three recipients of the SIIM 2018 New Investigator Travel Award.
A large dataset
Setting out to build a deep-learning algorithm that could ultimately be placed into clinical use, the researchers began by collecting a large database. Using natural language processing of reports and visual inspection of all the exams, Chang and colleagues searched for cases of hemorrhage among all 10,159 noncontrast head CT exams -- more than 500,000 images -- acquired between January and July of 2017 at their institution. Of these exams, 901 (8.9%) contained a hemorrhage, with 358 cases of intraparenchymal hemorrhage, 319 cases of epidural hemorrhage, and 224 cases of subarachnoid hemorrhage of various sizes.
Next, 3D voxel-level mask annotations were manually created for all of the cases with hemorrhage, and the data were fed into a mask-based residual 3D/2D CNN architecture for training to predict hemorrhage, Chang said.
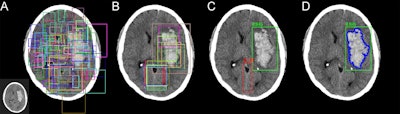
After fivefold cross-validation, the CNN yielded a high level of performance for detecting hemorrhage on the 10,159 exams. The algorithm missed only 26 (2.9%) of the 901 hemorrhages in the study, with similar performance across all sizes and types.
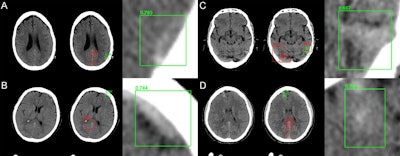
The mask residual CNN component of the deep-learning architecture can generate volume calculations of the hemorrhages that correlate highly with ground-truth measurements, according to Chang.
Clinical use
To facilitate clinical use of the algorithm, the researchers also developed a separate deep-learning tool that is highly accurate for determining CT acquisition parameters directly from image data. This facilitates end-to-end deployment of image analysis tools and removes reliance on underlying DICOM headers for determining which image series need to be reviewed by the algorithm, according to the researchers.
Since February 2018, the software tool has been used clinically for real-time interpretation of all head CT exams performed in the emergency room. Prospective data from one month of use showed that the algorithm identified 77 (95.1%) of 81 total cases of hemorrhage and yielded similar results to those achieved with the testing dataset.
Clinical performance of algorithm for brain hemorrhage detection | |
Accuracy | 97.2% |
Area under the curve (AUC) | 0.989 |
Sensitivity | 95.1% |
Specificity | 97.3% |
Positive predictive value | 97.2% |
Negative predictive value | 95.2% |
The research shows that real-world medical applications of deep learning can work, but they require a nontrivial set of circumstances such as customized network architectures, large representative datasets, clinical integration, and prospective testing, Chang said.
Future directions
The researchers have received a U.S. Food and Drug Administration (FDA) investigational device exemption (IDE) to fully integrate this algorithm into the radiology worklist.
"As we embark on this next stage, we're very actively looking for partners," Chang said.
He will continue working on this project as he assumes a new position in July as director of the University of California, Irvine's Center for Artificial Intelligence in Diagnostic Medicine (CAIDM).