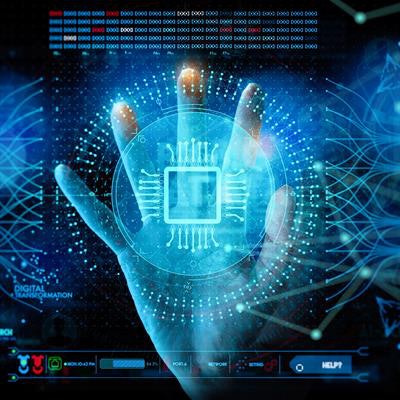
For a variety of reasons, functional and diffusion MRI haven't been particularly hot research areas for artificial intelligence (AI). But these advanced imaging modalities do offer a ripe opportunity, according to a presentation at the virtual 2020 American Society of Neuroradiology (ASNR) meeting.
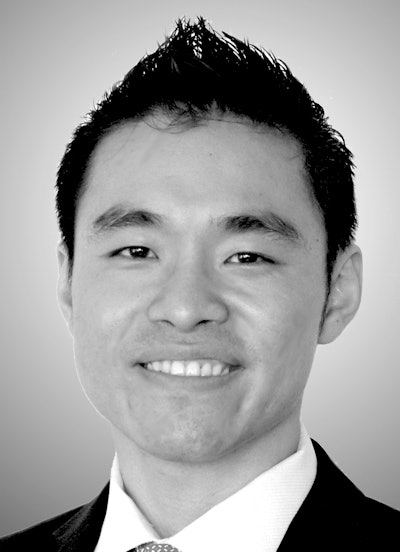
Deep learning could be used, for example, to enhance preprocessing, provide prediction or classification, improve signal decomposition, and bolster image reconstruction, according to Dr. Peter Chang of the University of California, Irvine. He spoke on the potential for AI as a tool for clinical translation of advanced diffusion and functional MRI in his talk.
"Advanced imaging with its very unusual and unique, densely sampled data does present unique challenges [for AI] and as a result does not tend to be studied with as much rigor and enthusiasm as perhaps some of the lower-hanging, easier fruit," he said. "But with that being said, there are quite a bit of some unique and interesting opportunities here."
Preprocessing pipelines
For example, many advanced MR processing steps could be augmented and/or replaced with deep-learning alternatives, according to Chang. These tasks include brain masking (extracting the brain from surrounding tissue on the image), tissue segmentation, and coregistration.
Deep-learning algorithms can offer speed and accuracy improvements in comparison with software tools currently in use, he said.
"A deep-learning tool is not only able to replicate these masks with greater speed -- on the order of seconds as opposed to minutes or hours for traditional techniques," he said. "The technique is also able to improve overall mask quality, especially at the edges or margins or extremes of the brain convexity where traditional models may struggle."
Predicting, classifying data
As functional imaging data is too dense and high-dimensional to be used efficiently on its own in deep-learning prediction models, the most salient features desired for the prediction task must first be extracted from the raw data and processed into a more useful format, Chang said.
"After you have that representation, you would then use those as inputs into some sort of a final predictive model," he said.
As an example of this technique, researchers from the University of British Columbia reported in 2017 that a 3D convolutional neural network that analyzed several imaging features extracted from functional and structural MRI data was able to outperform traditional machine-learning models for automatic diagnosis of attention deficit hyperactivity disorder (ADHD), Chang said.
Deep learning could also be used as the primary method for reducing the dimensionality of the raw image data. This can be achieved by deploying an autoencoder, a type of architecture that will first attempt to compress the original raw image into a finite number of inputs. The algorithm will then try to use this compressed raw image to recreate as much of the original image as possible, Chang said.
"It turns out that if you force the algorithm to do this somewhat unusual task, the intermediate feature vector -- the most compressed version of the data that you have -- turns out to be a really good, compact representation of all of the original data that you used to have," he said. "This itself, the feature vector generated by the autoencoder, can, in fact, be very useful for predictive tasks."
Researchers from China concluded in 2018 that such a deep discriminant autoencoder network could consistently improve the results of traditional techniques for classifying schizophrenia on functional connectivity MRI. Functional MRI correlation matrices were fed raw into an autoencoder to generate an intermediate feature vector, which was then used as an input for a deep-learning algorithm to predict schizophrenia, Chang said.
Researchers have found autoencoders to be useful in other instances as well, such as in developing algorithms for diagnosing autism on resting-state functional MRI and classifying Alzheimer's disease and mild cognitive impairment using MRI and PET data, according to Chang.
Signal decomposition
Signal decomposition may also be reformulated as a deep-learning problem, according to Chang. Although raw, densely-sampled data is very difficult for deep-learning models to deal with on their own, another option is to focus on very small numbers of pixels in that data and then analyze potentially interesting patterns, he said.
"In other words, I can take a raw acquisition, extract out the relevant temporal or time series data across this small [image] patch and try to predict something useful from that," he said. "[This is] very similar to a standard signal decomposition technique occurring voxel by voxel. But now, using a deep-learning technique, I am able to incorporate much more robust a priori assumptions into the model, and in addition, perhaps include more contextual information from the surrounding pixels."
For example, researchers have used this technique to estimate fiber orientations in diffusion imaging, Chang said.
Image reconstruction
In a related area, deep-learning algorithms can also be utilized in image reconstruction. Compared with traditional reconstruction methods, a deep-learning system may offer speed and performance improvements by being able to inject more a priori knowledge into the reconstruction task, Chang said.
For instance, a team of researchers in Germany reported in 2016 that deep-learning algorithms could reduce diffusion MRI processing from 12 steps to one, Chang said.