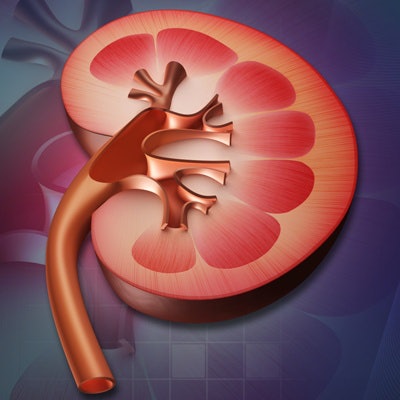
Artificial intelligence (AI) used with CT data could help abdominal radiologists better identify and classify kidney cancer, according to a presentation delivered at the recent virtual annual meeting of the Society for Imaging Informatics in Medicine (SIIM).
The detection of renal cell carcinoma has increased with the use of abdominal CT, presenter Chanon Chantaduly of the University of California, Irvine and colleagues wrote in their research abstract. In fact, in 2018, 400,000 patients around the world were diagnosed with the disease.
Chantaduly's group hypothesized that convolutional neural networks (CNNs) could improve the detection of renal cell carcinoma. The team used CT data from the 2019 Medical Image Computing and Computer Assisted Intervention (MICCAI) Kidney and Kidney Tumor Segmentation challenge (KiTS19) and patients from the university (210 MICCA KiTS patients and 109 Irvine patients). The Irvine patients underwent abdominal CT scans between 2011 and 2019 and received a renal cell carcinoma diagnosis.
Chantaduly and colleagues used data from 638 kidney scans in the total cohort of 319 patients, creating random training, validation, and test datasets. Training and validation datasets consisted of 269 patients, while the test set included 50 patients.
The group implemented two CNN algorithms:
- Bounding box localization of the right and left hemiabdomen. This algorithm consisted of three separate binary 2D CNN classifiers trained on sagittal, axial, and coronal orientations; its edges were the lateral, anterior, and posterior abdominal wall; the ipsilateral vertebral body margin; the inferior-most hemidiaphragm; and the superior pelvic wing. The algorithm's purpose was to cut out irrelevant information in the image.
- Segmentation of the renal parenchyma and tumor in each hemiabdomen. This algorithm was trained on the bounding boxes from the first algorithm, with right and left hemiabdomens cropped out.
The team evaluated the performance of the two CNN algorithms using the dice score coefficient (used to assess the similarity of two samples) and the Pearson correlation coefficient (which assesses the strength of the association between two variables). The closer the number of each of these measures is to 1, the more similar/strongly associated the variables are.
Chantaduly and colleagues found that these two CNN algorithms were effective for identifying renal parenchyma and tumors.
CNN algorithm performance for helping radiologists identify renal parenchyma and tumors | |||
Task | Dice score median deviation | Pearson coefficient for volume | AUC |
Renal parenchyma segmentation | 0.97 | 0.998 | 0.99 |
Renal tumor segmentation | 0.82 | 0.993 | 0.97 |
The two algorithms work together to improve CT images of the kidney, and therefore help radiologists make accurate diagnoses, Chantaduly concluded.
"The bounding box creates a more localized view ... [that allows the second algorithm] to produce a more specific segmentation of the kidney," he said. "In other words, there's more kidney and less irrelevant information."