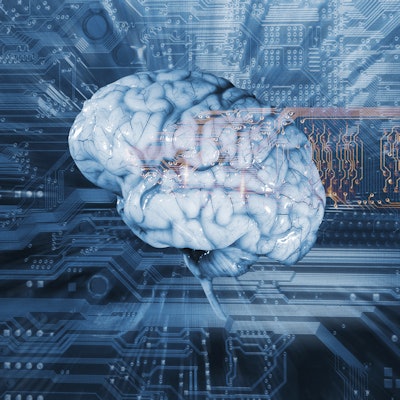
An artificial intelligence (AI) algorithm was able to segment and quantify hematoma on noncontrast whole-head CT scans in acute hemorrhagic stroke patients at an accuracy level comparable to that of an expert, according to research published online November 9 in Scientific Reports.
A team of researchers led by co-first authors Ali Arab, PhD, and Betty Chinda of Simon Fraser University in Burnaby, British Columbia, found that their deep-learning model outperformed three other previously evaluated machine-learning techniques and had similar performance to expert neuroradiologists. Also, it yielded segmentations much faster than previous machine-learning methods.
"The [deep-learning method] has the potential to be deployed in clinical settings in the future to assist physicians in identifying and evaluating hemorrhage and guiding clinical decision-making, leading to improved clinical outcomes for patients with hemorrhagic stroke," the authors wrote.
In hemorrhagic stroke management, detection, and quantification of the volume of hematoma is unequivocally important in several clinical scenarios, according to the researchers.
"First, in a triage system, such software tools can be used to identify the presence of hemorrhage in patients and alert physicians for immediate attention, reducing the turnaround time for patients with such critical conditions," the authors wrote. "Also, once a patient is diagnosed with hemorrhagic stroke, a physician can launch the software to obtain information about the volume and location of the hematoma, and consequently develop an effective intervention strategy. In addition, segmentation software can be useful in evaluating repeated imaging performed for hematoma growth estimation."
The researchers sought to develop a deep-learning model that would be useful as a potential software tool in all three of those clinical scenarios. They trained their automatic method, called convolutional neural networks with deep supervision (CNN-DS), using 45 randomly selected image sets from patients with acute hemorrhagic stroke across Fraser Health in British Columbia.
The images were acquired on several different CT systems, including Discovery CT750 (GE Healthcare), LightSpeed VCT (GE), and Somatom Definition Flash (Siemens Healthineers). Later, CNN-DS was tested on a separate test set of 10 image sets.
To create the ground truth reference standard, each CT scan was manually segmented slice by slice to provide a binary classification of hematoma versus normal brain tissue. These segmentations were performed by three raters, including two experienced neuroradiologists, as well as a neuroscience master's of science candidate whose work was double-checked by a third experienced neuroradiologist.
After training, CNN-DS yielded a Dice similarity coefficient of 0.88, higher than the 0.76 achieved by a different type of U-Net CNN. In terms of interrater agreement, CNN-DS produced a Cohen's kappa coefficient of 0.82 for hematoma quantification when compared with a human reader, less than -- but not statistically lower -- than the 0.88 achieved among the human raters.
Segmentation took an average of 0.74 seconds on a single graphics processing unit, according to the researchers.
"Further research is warranted to test the CNN-DS solution as a software tool in clinical settings for effective stroke management," they wrote.