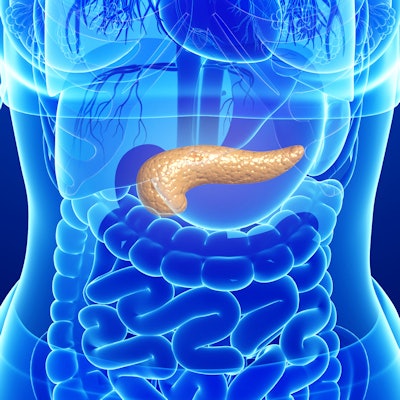
Using a deep-learning algorithm with CT can identify biomarkers that help clinicians detect type 2 diabetes in patients undergoing the exam for other indications, such as colorectal cancer screening, according to research presented at the recent RSNA meeting.
"We believe that CT can be useful to diagnose type 2 diabetes as it can provide information on pancreas morphology and is already widely used in clinical practice," presenter Hima Tallam told session attendees. Tallam is a medical and doctoral student at Rutgers New Jersey Medical School in Wayne, NJ. She conducted this research under the auspices of Dr. Ronald Summers, PhD's Imaging Biomarkers and Computer-Aided Diagnosis lab at the National Institutes of Health in Bethesda, MD; the research is part of a longstanding collaboration with Dr. Perry Pickhardt at the University of Wisconsin-Madison.
Tallam's group hypothesized that a nondiabetic pancreas would be smoother, denser, and larger than a diabetic one, and it would have less fatty infiltration. The team's study included 8,992 patients who underwent colorectal cancer screening with CT colonography between 2004 and 2016. Of these patients, 572 had type 2 diabetes and 1,880 were dysglycemic.
The researchers segmented images of the pancreas using a deep-learning algorithm that flagged biomarkers such as CT attenuation, pancreatic volume, intrapancreatic fat, and the fractal dimension of the pancreas. They also tracked the visceral fat, abdominal plaque, and peripancreatic plaque of the patients.
The deep-learning model showed that diabetics had lower pancreas CT attenuation and higher visceral fat than those patients who did not have the disease. The model also identified six predictors for type 2 diabetes, including the following:
- Intrapancreatic fat
- Pancreas fractal dimension
- Visceral fat volume
- Severity of plaque
- Body mass index (BMI)
- BMI higher than 30 kg/m2
Tallam's group found that the deep-learning model produced a pancreas segmentation Sørensen-Dice coefficient score of 0.69, the same as the interobserver Dice score. It also demonstrated a high intraobserver score of 0.81.
"The Dice score of 0.69 is considered state of the art for pancreas segmentation on noncontrast CT and was [the same as] interobserver score -- which demonstrates that person-to-machine performance is similar to person-to-person," she noted.
The fact that a condition such as type 2 diabetes can be identified on CT imaging for another indication could translate to better patient outcomes, Tallam said.
"[Our findings] could lead to future diabetes research investigating changes in pancreas morphology," she concluded. "We hope that it will inform diagnosis of early stages of type 2 diabetes and allow patients to make lifestyle changes to alter the course of the disease."
The research is part of a collaboration with Dr. Perry Pickhardt of the University of Wisconsin-Madison.