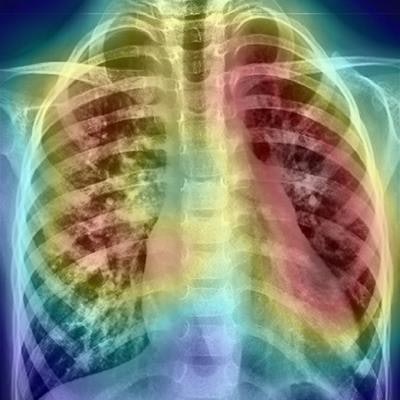
A deep-learning algorithm that analyzed chest x-rays was able to predict pulmonary to systemic blood flow just as well as clinicians. The finding could give healthcare providers a better way to determine which patients with congenital heart disease require intervention, according to a January 22 article in JAMA Cardiology.
A Japanese research team led by Dr. Shuhei Toba of Mie University developed a deep-learning algorithm based on convolutional neural networks (CNN) that analyzed chest radiographs, then compared its performance with that of human readers interpreting x-rays and using a conventional method for determining blood flow. The algorithm recorded higher accuracy, sensitivity, and area under the curve (AUC) than the human readers.
In introducing their model, Toba and colleagues noted the shortcomings of existing methods for determining the status of pulmonary to systemic blood flow in patients with congenital heart disease -- a key step in deciding which patients would benefit from surgery or cardiac catheterization. Echocardiography can only be used in patients with simple cases of heart disease, while cardiac MRI has drawbacks due to its use of magnetic fields and the length of exam time.
Chest radiography is commonly used to assess pulmonary blood flow status, but the evaluation of images is subjective and not quantitative. Could deep learning help out?
To that end, the researchers developed a CNN deep-learning algorithm and tested it in 657 patients. They then compared its performance with that of human readers in a subgroup of 78 randomly selected patients who received a total of 100 cardiac catheterization procedures.
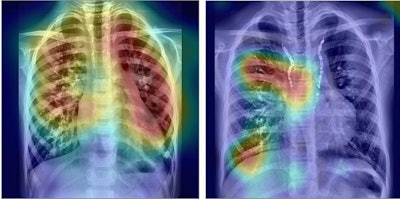
The human readers included pediatric cardiologists and pediatric cardiology fellows who analyzed pulmonary blood flow using the Fick method, a method for calculating blood flow to an organ using a marker substance. Patients were characterized into one of four groups based on low to very high blood flow.
The algorithm correctly classified 64 of the 100 chest radiographs, versus 49 of 100 for the human readers, the researchers found. When it came to detecting a pulmonary to systemic blood flow ratio in the "high" category, the algorithm performed as follows:
Performance of deep learning for detecting high pulmonary to systemic blood flow ratio | |||
Fellow readers | Expert human readers | Deep-learning algorithm | |
Accuracy | 0.78 | 0.80 | 0.86 |
Sensitivity | 0.11 | 0.16 | 0.47 |
Specificity | 0.94 | 0.95 | 0.95 |
AUC | 0.67 | 0.78 | 0.88 |
"In terms of classifying chest radiographs into four classes depending on pulmonary blood flow, our study showed that deep learning-based analysis outperformed the clinicians whose diagnostic performance was correlated with their level of experience," Toba and colleagues wrote.
The researchers further postulated that their deep-learning model had the capability to help physicians justify interventional in cases of congenital heart disease. However, they also noted that the physicians in the study were not provided with clinical information such as a diagnosis or past medical history that would be available in a real-world setting, and, thus, their study might have underestimated the performance of the human readers.